Digital Health
4 Roadblocks Hindering The Promise of Predictive Analytics
Brad Eckler, Field Sales Director, Public Sector, Qlik COVID-19 will likely impact and strain healthcare systems for many years to come — possibly even…


COVID-19 will likely impact and strain healthcare systems for many years to come — possibly even forever. As its attention wanes, we need technology to step in and tell us when and how to act next. What if there was a way to predict the next COVID-19 surge months before it affected operations? How many beds will be available? PPE? Where will you need to allocate additional resources and staff? What other seasonal uptick of disease will it align with? Will our revenue take a loss?
These questions can be turned into real actionable answers through the power of predictive data analytics.
Healthcare systems are flooded with big data — large, complex, and high-velocity datasets from many sources, from patient health data to real-time operations data and claims data. Predictive analytics not only helps manage but also capitalize on this influx of data, to make better, data-driven decisions that can improve overall performance. Healthcare organizations now have the opportunity to better arm themselves and take a proactive approach in navigating their toughest challenges – whether it be a global pandemic or simply day-to-day operations.
By building upon descriptive and diagnostic analytics — the “what happened?” and “why did it happen?” — we are able to use statistical modeling, data mining, and machine learning to deploy predictive analytics — and better answer the question “what will happen?” Both also provide a foundation for prescriptive analytics, in which we are able to make specific recommendations on the best course of action based on current and historical data. These predictions help guide leadership decision-making to mitigate risk, improve efficiency, and identify opportunities or allocation. Additionally, it lowers the risk of human bias or error, as decisions are driven by data, not instinct.
In one case study, Appalachian Regional Healthcare, serving rural communities of West Virginia and Kentucky, was enduring a high frequency of missed appointments. This raised concern for the not-for-profit’s revenue, but more importantly, the patient’s health — especially with the region’s prevalence of chronic issues. To address the issue of no-shows, ARH opted to pursue a machine learning (ML) solution to identify patterns in historical data and to predict future results based on those patterns. Examining the information on patients who had previously missed appointments, such as what health conditions they had, or demographic information like age and sex, they then could use any patterns identified by the model to identify similar patients. By addressing the signals of appointment cancellations identified by the ML model, ARH reduced cancellations and no-show rates at their pilot clinic from 20 percent to 15 percent over a three-month period.
Additionally, in a recent survey conducted by Syntellis Performance Solutions, 46 percent of healthcare finance executive respondents stated that they want to implement predictive analytics to better determine the best path forward for patients and providers. Though the value proposition is clear and very favored by executives, why is this technology not being utilized in every healthcare system? Short answer – there are several roadblocks that are slowing implementation.
1. Data Illiterate Workforce. According to a survey conducted by Qlik, only 32 percent of C-suite leaders are viewed as data literate, potentially holding senior leaders back from encouraging their workforces to use data to their advantage. Leaders within healthcare systems may not have the skills to support predictive analytics. Users may require in-depth data literacy training and proper onboarding to fully understand and utilize the technology.
2. Lag in Cloud Migration. Cloud platforms can help organizations strive for more data-driven patient care, while also focusing on patient care and security. However, organizations that are lagging in their migration to the cloud may also miss out on tapping into the full power of predictive analytics. Many development efforts are geared toward the cloud, putting organizations that are still heavily on-prem — mostly due to HIPAA or other privacy obstacles — at a disadvantage.
3. Interoperability Issues. Interoperability is the key to unity among multiple functions across healthcare organizations. The lack of data interoperability in healthcare can cause clinical, financial and other data sets to reside across different systems and in different formats. Because of this, interoperability may need to be addressed and improved before predictive analytics can be implemented.
4. Overcoming Algorithmic Bias. Artificial intelligence has long suffered from algorithm bias and there is fear that it has the potential to further exacerbate social inequities. To ensure predictive analytics does not do more harm than good, its data needs to be mindful of these potential biases. It is necessary to identify and address certain demographic groups that are disadvantaged and work to “protect” them so that their needs are not overlooked by the algorithms.
Though overcoming these barriers may seem like a daunting task, the value that predictive analytics brings is extremely promising. As healthcare organizations continue to overcome these challenges and strive toward modernization, they should seek more expert research and investment in new data-driven solutions that will ultimately transform the future of care delivery.
About Brad Eckler
Brad Eckler is the Field Sales Director of Public Sector at Qlik a data-literate world, where everyone can use data and analytics to improve decision-making and solve their most challenging problems.
artificial intelligence
big data
machine learning
data analytics
healthcare
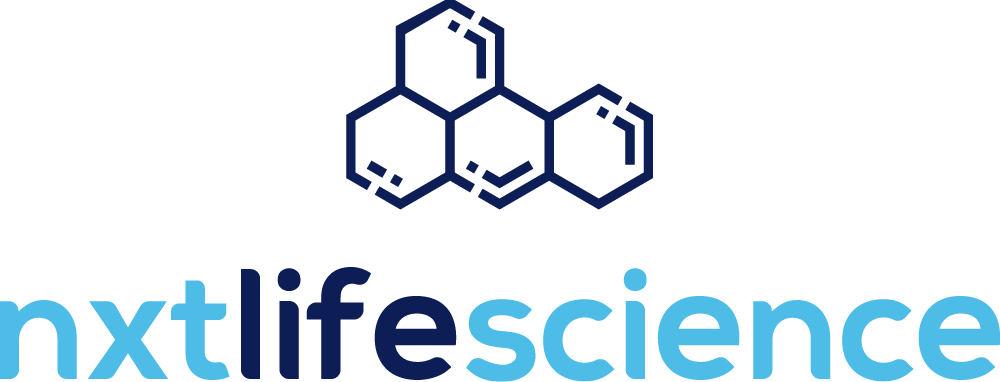
Keep it Short
By KIM BELLARD OK, I admit it: I’m on Facebook. I still use Twitter – whoops, I mean X. I have an Instagram account but don’t think I’ve ever posted….
Asian Fund for Cancer Research announces Degron Therapeutics as the 2023 BRACE Award Venture Competition Winner
The Asian Fund for Cancer Research (AFCR) is pleased to announce that Degron Therapeutics was selected as the winner of the 2023 BRACE Award Venture Competition….
Seattle startup Olamedi building platform to automate health clinic communications
A new Seattle startup led by co-founders with experience in health tech is aiming to automate communication processes for healthcare clinics with its software…