Digital Health
5 Ways Federated Computing Can Reshape Public Health
Unlocking data silos using Federated Computing (FC) has the potential to achieve a positive impact across the healthcare industry, ranging from clinical…


Unlocking data silos using Federated Computing (FC) has the potential to achieve a positive impact across the healthcare industry, ranging from clinical care quality improvement and accreditation to population health management, precision public health and equitable drug development.
We define FC as the leverage of federated learning and edge computing technologies to enable federated data access, enabling analytics and Artificial Intelligence (AI), using linked, but not co-located, data. In turn, the superior privacy posture offered by this approach is appealing to data owners as it lets them remain in control of their data at all times, and thus reducing their administrative overhead and increasing their willingness to participate in data-driven innovation.
By leveraging this emerging technology, innovators and AI developers can enjoy more diverse data to develop AI models that help drive positive transformation of the industry, while data owners who may not have the resources to develop AI models still get the opportunity to participate in- and access innovation. The same holds true for large-scale quality improvement efforts, which typically are held back by the challenges of accessing and analyzing data from large networks of providers, making them costly to participate in. Based on early signals from our partners and from the broader ecosystem, we anticipate that the effects will be most palpable across five primary segments (all depicted below).
1. Enabling Large-Scale Quality Improvement- and Accreditation Efforts
The strong privacy posture offered by privacy-preserving, distributed analysis compared to direct data sharing makes it easier for healthcare providers to participate in quality improvement efforts by lowering barriers to scalable quality benchmarking. This is accomplished by reducing the administrative burden and cost for providers (e.g., data sharing agreements, compliance), as well as by lowering data privacy risks. In addition, capabilities to run data harmonization and other operations to prepare data for analysis reduce the intensity of project management and coordination needed to orchestrate efforts across sites (which otherwise tends to increase exponentially in complexity as these networks scale).
FC has a role to play in this ecosystem by allowing high-quality centrally defined risk adjustment models and quality metrics to be developed and validated in broader settings, ensuring a fair and unbiased assessment of quality, as well as by being more readily deployed and executed at individual provider sites without direct data sharing. In practice, using FC, high-quality risk adjustment algorithms and comprehensive quality metrics can be developed and validated by leading quality assessment entities, including accreditation agencies like the American College of Radiology (ACR) or bodies that develop, validate and measure quality, like the National Quality Forum (NQF) or the International Consortium for Health Outcomes Measurement (ICHOM), to be subsequently applied to assess the quality of care across multiple healthcare providers.
The resulting improved transparency on quality of care then allows for identifying best practices for others to learn from and is likely to attract the interest of public funding bodies who are increasingly interested in reimbursing for value. By linking reimbursement to care quality, the healthcare system can adopt a more value-based approach, triggering a virtuous cycle where healthcare providers are primarily incentivized towards improving patient health. Different entities are already investing significant efforts in comparing health outcomes and various process metrics across healthcare providers, but given the complexities of execution, these are often limited in scope and require much time to execute.
By making it easier to obtain transparency on quality, FC thus has an important role to play in establishing this value-based care ecosystem. Beyond enabling value-based reimbursement, the resulting transparency can exert a positive impact by helping fuel a palette of applications that empower patients to make more informed choices about their care, much like what is observed in other industries, where platforms for comparing price and performance of products and services are commonplace.
2. Enhancing Population Health Management
Improved population health management (PHM) capabilities can be achieved by effectively disseminating more generalizable patient risk segmentation models, to identify individuals at high risk of experiencing poor health outcomes. FC will let leading developers create robust analytical approaches and risk segmentation models, trained on data from diverse populations, which can then be distributed to healthcare providers that may lack the expertise to develop such models themselves.
By identifying patients at high risk of poor health outcomes in advance, these risk segmentation models enable timely interventions, such as primary and secondary preventive care, resulting in improved patient outcomes. As data remains within the data custodian’s control and behind their firewalls, FC reduces the risk for individual institutions to participate in PHM efforts, driving wider dissemination as well as making data from more diverse populations available for model training. In turn, this will improve the generalizability and performance of the models, which translates to an increased ability to find the patients who need intervention before it becomes too late.
3. Syndromic surveillance
In addition to creating data silos that impact the external validity of insight and the generalizability of models, the current barriers to accessing and deriving insights from data across a network of institutions severely impact the ability to identify signals of emerging epidemics. Given the often rapid spread of contagious disease, it is important that data can be accessed and analyzed as early as possible when disease spread begins, to enable public awareness and intervention. Horror stories from the recent COVID-19 pandemic depicted how medical students were commissioned by the hour to manually abstract data from unstructured EHR notes into an array of disease registries, which came at a high cost and with a data velocity that was not conducive to tracking pandemic spread in a manner suited for timely intervention.
To ensure that the next pandemic is detected in time for institutions to prepare and monitor in a manner that allows for successful intervention, ideally avoiding some of the strict lockdown restrictions from a few years ago, governmental agencies should consider investing in developing a distributed network that allows for near real-time access and analysis of real-world data. The need has already been recognized at the grassroots level, as is for example illustrated by networks of hospitals that collaboratively work to harmonize data and make it useful for collaborative analysis. However, the computational infrastructure for operationalizing such data without the need for transferring it across institutions remains to be implemented.
4. Advancing Precision Public Health
While FC clearly can help advance traditional precision-medicine use cases such as enhancing response rates to medicines by enabling AI models that better match patients with the appropriate therapy, it also has a role to play in conjunction with the future of public health. In particular, the emerging paradigm of precision public health (PPH) holds promise to better target public health efforts within populations, to ensure effective and equitable allocation of funds as precision medicine gradually will transform care delivery models.
While it is well known that not all individuals in a population respond equally well to a given therapy, e.g., due to differences in genetic makeup that impact metabolization patterns, or due to concomitant medical conditions, the standard approach to finding a treatment that works is based on doctors iterating through different treatment approaches. In turn, this trial-and-error approach poses risks to patients and also drives cost, as patients tend to receive (costly) medications that may not be safe or effective for them before settling on one that is. In the future, recommendations from AI models trained on patient data can help doctors narrow down treatment options to those that are expected to work and thus support them in making safer, more effective and less costly choices for their patients. This benefit of moving away from the ‘one size fits all’ paradigm holds for public health initiatives as well, as not all interventions will be equally effective for everyone, thus driving low value care in certain populations.
While the direct physical- and financial risk resulting from subjecting a patient to an ineffective public health intervention may be lower than subjecting them to an ineffective medication, we expect that better allocation of public health interventions will translate to better overall cost containment in the healthcare system, as well as to improved patient outcomes. In this context, FC again acts by lowering barriers for individual institutions to participate in the development of models for assessing the likelihood of an intervention (e.g., a public health program) being effective in a given population, thus leading to greater diversity of the data available for model development and as a result to improved model performance.
5. Enabling Equitable Drug Development
In addition to the challenges of matching the right patient to the right intervention, the healthcare industry struggles with inequitable care in the context of life sciences innovation that insufficiently takes into account traditionally disadvantaged populations. This largely comes down to the fact that executing development programs and clinical trials in these populations is sometimes challenging due to a number of reasons not all elaborated here. While this pain point has caused real-world data (RWD) vendors and related service providers to increasingly take patient diversity into account, the footprint of these platforms is still largely confined to the sites and populations that tend to be well represented in clinical development programs already.
By reducing the data privacy risk for clinical sites to participate in pre-screening and trial site-selection endeavors, FC can help lower the barriers for non-traditional sites to participate in clinical development. In practice, this would let them swiftly undertake feasibility screening using algorithms that have been developed elsewhere, in a secure manner that does not involve any data transfer to a third party. The larger the number of diverse clinical sites that make their RWD available to the life sciences industry for such pre-screening, the more effective trial site allocation becomes and the more likely it is that trials can be completed on time and in a diverse enough population to make them externally valid.
Beyond helping to ensure that medicines are developed for everyone, broader geographical coverage can help life sciences companies identify clinical sites that may have been overlooked previously, but that have a high potential for successful patient recruitment and limited competition from biopharma peers. Finding such sites promises significant savings in terms of time and effort, and can lead to shorter-term returns on investments in the proposed federated infrastructure.
About Mathias C. Blom
Mathias C. Blom (MD, PhD, MSc) is the Partnerships Vice President at Rhino Health. As a Lund University and Harvard graduate, Mathias’ career has focused on innovating and advancing the technologies shaping the future of healthcare. From developing machine learning pipelines to leveraging predictive analytics for accelerating innovation in healthcare applications and medical research, Mathias is passionate about making a positive change in all life sciences sectors to improve patient care.
artificial intelligence
ai
machine learning
healthcare
medicine
medical
public health
therapy
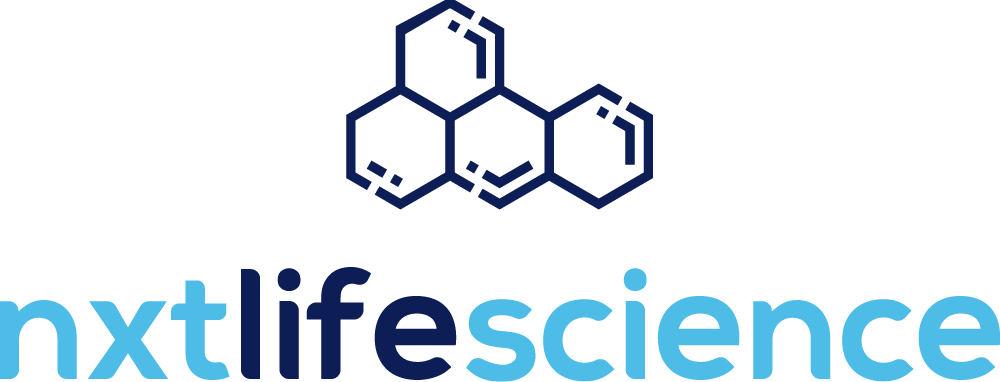
Keep it Short
By KIM BELLARD OK, I admit it: I’m on Facebook. I still use Twitter – whoops, I mean X. I have an Instagram account but don’t think I’ve ever posted….
Asian Fund for Cancer Research announces Degron Therapeutics as the 2023 BRACE Award Venture Competition Winner
The Asian Fund for Cancer Research (AFCR) is pleased to announce that Degron Therapeutics was selected as the winner of the 2023 BRACE Award Venture Competition….
Seattle startup Olamedi building platform to automate health clinic communications
A new Seattle startup led by co-founders with experience in health tech is aiming to automate communication processes for healthcare clinics with its software…