[To share your insights with us, please write to sghosh@martechseries.com]
Digital Health
AiThority Interview with Yan Chow, Global Healthcare Leader at Automation Anywhere
The post AiThority Interview with Yan Chow, Global Healthcare Leader at Automation Anywhere appeared first on AiThority.



Hi, Dr. Chow. Please tell us a little bit about your role and responsibilities in your current company. How did you arrive at Automation Anywhere?
I joined Automation Anywhere nearly four years ago to launch and lead the healthcare verticaland, later, the life sciences vertical as well. My goal is to help move healthcare toward a sustainable, scalable 21st century care delivery model. My background includes both healthcare and life sciences, and my career has been at the intersection of medicine, technology, innovation, and business.
Prior to Automation Anywhere, I was a practicing physician for several decades at Kaiser Permanente, the largest private integrated care system in the U.S., then I moved into their national innovation leadership team. After that, I became chief innovation officer at a federal IT consulting firm, then I led digital medicine at Amgen, a leading biotech company.
What are the critical challenges impeding the growth and distribution of AI in the healthcare industry?
AI has made significant inroads into healthcare in niche areas such as diagnostic imaging, where its value is clear. However, there are considerations that remain to be addressed to broaden the use of AI in the healthcare industry, including:
- Access to good data. The accuracy and completeness of data are crucial for developing effective AI models in healthcare. However, healthcare data is often incomplete, inconsistent, unstructured, and spread across different systems. HIPAA concerns make it difficult to access and share patient data. Many healthcare organizations still struggle with legacy data integration, quality, and accessibility. This can result in incomplete or inaccurate data, which hurts the performance of AI algorithms.
- There are no standard protocols for the development and validation of AI models in healthcare. This can lead to inconsistencies in AI model development, making it difficult to compare results across studies and across time. In general, the healthcare industry is still early in standardizing data formats, collection, terminology, and clinical workflows. This makes it challenging to develop AI algorithms that can be applied across different healthcare systems.
- The healthcare industry is heavily regulated from top to bottom. The regulatory requirements for approval and implementation of AI-based solutions are still evolving, making it difficult for healthcare organizations to invest in, fully adopt, and implement these technologies. Issues include the nature of explainability in black-box systems, observational studies vs. randomized controlled trials, and hidden bias.
- Privacy and security. Patient privacy and data security are critical concerns. Healthcare organizations must ensure that patient data is kept secure and only used for authorized purposes. AI can create privacy and security breaches at scale if misused or used without safeguards. The massive data sets leveraged can be hacked, and AI itself can be designed by a bad actor to re-identify anonymous patient data.
- Ethical and legal issues. The use of AI in healthcare raises ethical and legal concerns, such as data privacy, bias, discrimination, transparency, accountability, and the potential for AI to prematurely replace human judgment and decision-making. AI errors and misuse can lead to serious consequences, thus consensus-driven ethical and legal frameworks are needed to guide development and implementation.
- AI development and implementation can be costly in terms of financial, infrastructure, and people investments. There will be concerns about the return on investment over time. Additionally, the cost of implementation can be a barrier for smaller healthcare organizations or those in under-resourced countries. Even though most agree AI will be an essential part of future healthcare, the ideal path to that reality will vary among healthcare organizations based on financials.
- Resistance to change. The healthcare industry is notoriously slow to adopt new technologies, for understandable reasons. Minimizing financial, patient, and regulatory risks is a sine qua non in healthcare. But sometimes, healthcare providers may be hesitant to use AI-based solutions due to a lack of understanding, fear of job loss, or concerns about the impact on patient care.
Recommended: AiThority Interview with Malcolm Koh, Director, CX Practice at Zendesk
Could you tell us about the role of AI technology leaders in helping healthcare organizations monetize their AI investments?
Given the competing priorities most healthcare organizations have, it is imperative that an AI technology leader (AITL) discuss with senior leadership the role of AI, possibilities for near-term monetization, and the strategic return on investment over the long term. In healthcare, AI can improve not just revenue and cost savings but also quality of care, regulatory compliance, patient experience, supply chain, and many other areas across the organization. Excellence in these areas is often just as valuable as financial returns.
To develop a compelling business case for AI, an AITL will need to understand the healthcare landscape. A deep understanding of the industry—including its regulatory environment, market trends, and patient needs—is needed to identify AI solutions that can generate revenue nearterm and longterm while aligning with business objectives. A short-term, clearly scoped win that delivers on its promises can help open the gates to broader enterprise-wide adoption.
Knowing what AI can and cannot do helps the AITL define the correct KPIs, especially if an early project is to drive, accelerate, or recover revenue. The AITL will want to monitor, measure, and analyze impact on KPIs like revenue, costs, and patient outcomes, while tracking the success of AI initiatives over time.
To ensure success, the AITL must collaborate with business or clinical colleagues within resource constraints, select appropriate technologies, develop customized algorithms, and integrate AI solutions into existing workflows. The AITL will want the best chance of success by ensuring data quality, integrity, completeness, and accessibility. They must also be careful not to break any of healthcare’s rules and regulations. Given the nature of healthcare, senior leadership is often more concerned about potential risk than projected benefit. AI solutions must comply with regulatory requirements like HIPAA and healthcare industry standards. Patient data must be protected, and AI solutions must be developed and implemented in as ethical and responsible a manner as possible.
The US Chamber of Commerce recently announced it is looking to establish ethical AI and tech-neutral AI laws for the future. What are your views on pushing the barriers in ML infrastructure using ethical means?
The U.S. Chamber of Commerce recently released a report calling for a risk-based regulatory framework that incorporates ethical safeguards.
The report developed five important principles and accompanying recommendations: (1) review and update of current regulation; (2) collaboration across federal agencies; (3) neutrality and a focus on outcomes rather than technologies, allowing a flexible, industry-specific approach; (4) allowing more agile, flexible private sector approaches to risk management and innovation due to the pace of technology; and (5) risk-based evaluation of any regulatory gaps that leads to a balanced and appropriate approach.
While I agree wholeheartedly with the Chamber’s thoughtful review, I see real challenges in two areas that can be illustrated with the latest trend, generative AI.
The first challenge is technical. Even in the private sector, the technology will quickly outpace existing regulatory, legal, and ethical guidelines, as it often does. A good example of this is ChatGPT, which was ready for deployment in early 2022, but not released until November of that year. Why? The development team spent more than eight months trying to establish guardrails so that the technology, trained on the broad internet, would not produce illegal, racist, and otherwise offensive output in inappropriate settings. Their efforts helped, but did not totally fix the issue. This example shows the tremendous difficulty of truly regulating a highly complex technology that if filtered would be less useful for certain applications, but if unfiltered, intolerable for others.
The second is enforcement. ChatGPT was the first generative AI to become mainstream due to the ease with which lay people could try it, garnering over a million users within one week of release. But it is only one among many generative AI engines, both commercial and research. Recently Stanford announced a competitive open-source version that actually exceeds ChatGPT’s performance in some areas. There are dozens of such research developments in the U.S and in other countries, and it will be impossible for the U.S. to regulate all such technologies. In this new ‘cold war’, we may ultimately end up in an arms race where we must use AI technologies to identify and combat rogue Ais, much like computer viruses. Regulation alone will be insufficient without technological weapons.
Recommended: AiThority Interview with Shaun McGirr, Field CDO at Dataiku
Please tell us more about AI’s role in relieving economic pressure and transforming healthcare services. Any particular example you would like to share with us from recent developments?
AI can be used to improve patient experience and outcomes, increase operational efficiency and cost savings, improve claims accuracy and write-offs, and accelerate revenue capture.
For instance, it can be trained on incredible amounts of knowledge—much more than one human could digest and recall in real time—which can help the healthcare providers who use it make more informed diagnoses and effective treatment recommendations.
To help illustrate this, social determinants of health (SDOH) have an outsized impact on health and quality of life. Demographics also play a role in how patients prefer to receive and then respond to diagnoses, including whether they follow through with a course of treatment. By taking SDOH and other demographics into consideration, AI can help doctors determine the best way to communicate diagnoses, prescribe treatment in a way that patients understand and are more likely to adhere to, and encourage better patient outcomes.
Tech companies are introducing ChatGPT3-based tools and capabilities for various business processes. What are your thoughts on this blazing trend?
I am excited by the various applications of AI-based tools and capabilities being introduced in business, and especially in the healthcare industry. ChatGPT can be integrated into the electronic health record (EHR) system to assist with the data input process. As discussed previously, it can support the process of making accurate diagnoses and treatment recommendations. It can also facilitate better telemedicine experiences to provide patients with technical support and medical information—a more informed replacement for online search, many patients’ go-to resource for initial medical advice.
An additional area of potential for ChatGPT is in simulations. A physician in medical school or their residency may see value in setting up a simulation via ChatGPT to practice communication with different patients. For example, a physician may encounter a particularly combative patient in their career, and a ChatGPT simulation might help the physician understand the best way to engage with them. Or a physician may be working in a different country than they were raised in. A ChatGPT simulation could help get them up to speed on the unique cultural norms and expectations that they should consider in discussing signs and symptoms, diagnosis, and treatment with their patients.
How could technology platforms benefit from leveraging Generative AI?
From my perspective, technology platforms and their users will get the most from leveraging generative AI when done so in combination with robotic process automation (RPA). Generative AI is the brain, providing ideas and direction, while automation is the arms and legs that carry out the work. To continue with the anatomical metaphor, they truly do go hand in hand.
In the healthcare system, this combination could help address some of issues surrounding unpredictable demand and labor shortages. We are nowhere near the possibility of workers being replaced by AI. In contrast, AI can greatly enhance the execution of uniquely human skills like empathy, creativity, problem-solving, and judgment.Most healthcare AI programs are designed to empower and augment the highly skilled healthcare worker.
Also, more broadly speaking, I find it interesting how generative AI is a user-friendly interface that, at an early stage, can help machines understand users much better than users understand machines. For decades, machines have gathered data on humans and human behavior—and they can now tap that data to make it easier for humans and machines to work together. I’m excited to see how this continues to evolve.
Your take on the future of ML uses in healthcare and finance:
Although healthcare certainly has traditional finance departments with responsibilities like tax planning and accounting, a compelling sweet spot for AI, machine learning, and RPA is the revenue cycle.
The revenue cycle essentially follows a patient through the process of obtaining healthcare, from the point when they pre-register for an appointment to when a bill is sent. It’s a very well-defined processthat AI, machine learning, and RPA can simplify and accelerate throughout.
From the start, these technologies can help a receptionist or scheduler understand the patient’s circumstances. They can support physicians and other staff in understanding why a claim was denied by insurance, then facilitate the development of an effective denial claim to ideally achieve a better outcome. They can help physicians obtain prior authorizations for procedures. They can even help patients better understand the bill that they receive following an appointment.
Many healthcare providers are not currently automating this process. A 2022 survey by AKASA found that around a quarter of healthcare finance leaders say they need to hire more than 20 employees to fully staff their revenue cycle departments. Not being able to adequately hire for this manual process is a significant problem for healthcare organizations—presenting a strong opportunity for AI, machine learning, and RPA to make the most of existing staff and resources.
Recommended: AiThority Interview with Lori Anne, Director of Product Development & Management at Verizon
Thank you, Yan! That was fun and we hope to see you back on AiThority.com soon.
Yan Chow, MD, MBA is the Global Healthcare Industry Leader at Automation Anywhere, a leader in the robotic process automation (RPA) industry. Previously he served as Medical Director for Digital Medicine in the Translational Medicine group at Amgen, where he led efforts to incorporate digital technologies and advanced analytics into clinical trials. Prior to that, Dr. Chow was Chief Innovation Officer at LongView International Technology Solutions. Until 2014, Dr. Chow was the national Director of the Innovation & Advanced Technology Group (IAT) at Kaiser Permanente and assessed emerging clinical information technologies; Dr. Chow also had a successful clinical practice at Kaiser Permanente for several decades. Dr. Chow has founded and advised several startups in the healthcare technology, storage, and database spaces and has received multiple awards for his work in healthcare IT. He holds an A.B. from Harvard University, an M.D. from UC San Diego, and an MBA from UC Berkeley’s Haas School of Business.
Automation Anywhere is the No. 1 cloud automation platform, delivering automation and process intelligence solutions across all industries to automate end-to-end business processes for the fastest path to enterprise transformation. The company offers the world’s only cloud-native platform combining RPA, artificial intelligence, machine learning, and analytics to automate repetitive tasks and build enterprise agility, freeing up humans to pivot to the next big idea and build deeper customer relationships that drive business growth.
The post AiThority Interview with Yan Chow, Global Healthcare Leader at Automation Anywhere appeared first on AiThority.
artificial intelligence
ai
electronic health record
telemedicine
machine learning
healthcare
medicine
medical
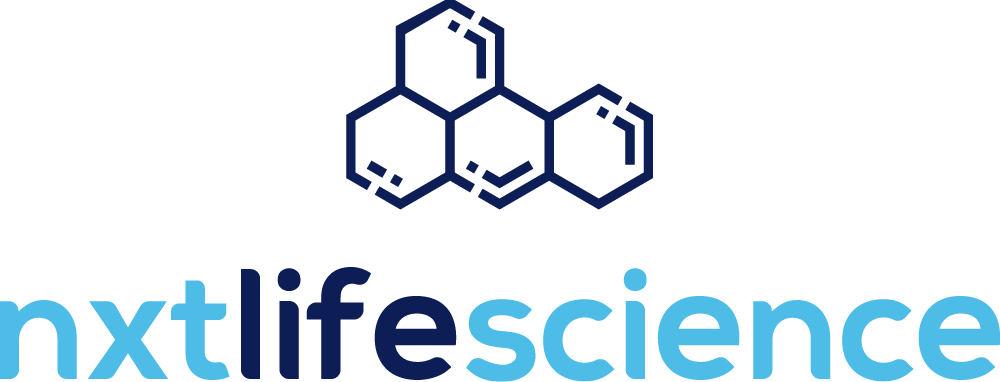
Keep it Short
By KIM BELLARD OK, I admit it: I’m on Facebook. I still use Twitter – whoops, I mean X. I have an Instagram account but don’t think I’ve ever posted….
Asian Fund for Cancer Research announces Degron Therapeutics as the 2023 BRACE Award Venture Competition Winner
The Asian Fund for Cancer Research (AFCR) is pleased to announce that Degron Therapeutics was selected as the winner of the 2023 BRACE Award Venture Competition….
Seattle startup Olamedi building platform to automate health clinic communications
A new Seattle startup led by co-founders with experience in health tech is aiming to automate communication processes for healthcare clinics with its software…