Digital Health
Evaluating Evidence Base in Digital Health Solutions: The Real Questions Stakeholders Should Be Asking
Siobhán Harty, Digital Health Scientist at SilverCloud Health, a part of Amwell Throughout the pandemic, digital health companies have seen significant…
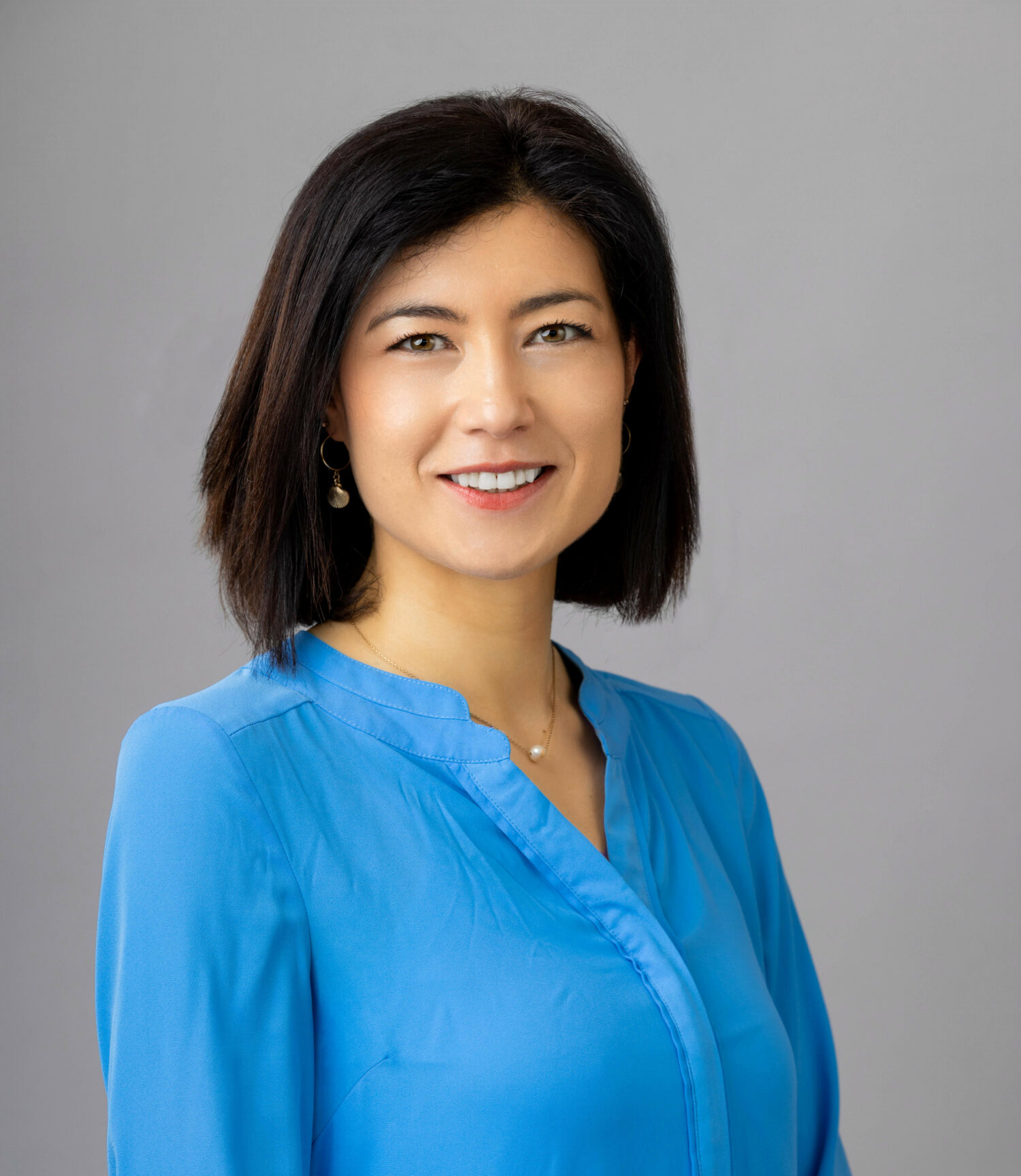

Throughout the pandemic, digital health companies have seen significant growth, with one study finding that U.S.-based digital health startups surpassed $29 billion in 2021, almost twice the funding seen in 2020. When done correctly, the use of these digital innovations can help people reduce the burden of dealing with mental health challenges by providing accessible, affordable, and timely care to users. As digital mental health interventions (DMHI) continue to battle it out for a leading spot in this growing market, it’s imperative for stakeholders like employers, health systems, and health plans to carefully evaluate each available solution.
Unfortunately, many digital mental health companies make false promises to their users, backing up their solutions with misleading claims that are not clinically validated and rooted in evidence-based research. A recent review of 293 apps for anxiety and depression found only 6% of companies claiming to use evidence-based frameworks in their product descriptions had published evidence supporting their effectiveness. Related research has also revealed that there is no correlation between app store metrics, such as star ratings and downloads, and more clinically relevant metrics relating to effectiveness and engagement.
This lack of correspondence between the popularity and market value of products and the strength of evidence on their effectiveness has been observed throughout the digital health field. For instance, a systematic review of the published research output from healthcare technology unicorns revealed that the highest-valued health tech startups had limited or non-existent supporting peer-reviewed evidence. Instances like this not only hurt the future and reputation of the digital healthcare industry but also expose risk to vulnerable users who are in desperate need of effective mental healthcare. When it comes to stakeholders, building mutual trust is a critical move, while also ensuring digital healthcare tools and platforms provide seemingly effortless access and promote the best quality of patient care. Digital health tools are growing in popularity, but if false evidence and claims are presented to users, we could see more and more people turn their heads on the future of this important market.
Below are five essential questions stakeholders should ask when evaluating the evidence base of digital mental health intervention:
1. Is the product truly evidence-based or merely “evidence-informed”?
One thing stakeholders need to ask themselves when evaluating digital health tools is whether the solution is truly evidence-based or just “evidence-informed.” A vast majority of DMHI in the market today promise to have evidence-based claims or clinically proven research but are only actually “evidence-informed”. To break it down, many company products are relying on evidence from other companies or even other researchers instead of conducting their own well-designed studies. “This form of extrapolation does not hold up well for DMHI, where the functionalities of the digital platform and the specific context surrounding the therapeutic content and are often a core part of the intervention frame and can fundamentally influence the potential for therapeutic benefit.
” Fidelity to even the core principles of well-established evidence-based treatments is rare among “evidence-informed” products. For instance, a review of 100 DMHI proposing to offer behavioral activation (BA) or cognitive behavioral therapy (CBT) established that only 10% had features consistent with the core principles of BA and CBT. One, particularly concerning consequence of this poor fidelity to established evidence-based treatments, is that users of these technologies can be misled to believe that they have tried CBT or BA and may be discouraged from exploring it again as they believe they have determined it does not work for them.
One way for stakeholders to be confident that a product is evidence-based as opposed to merely evidence-informed is to determine that the company has conducted a direct evaluation(s) of its product through well-designed research trials. Stakeholders should also verify that the claims the company is making about their product are substantiated by the evidence from those direct evaluations as opposed to the ‘evidence’ being misrepresented in a manner that does not reflect the true conclusions that can be drawn from the research trials.
2. What type of research trials were employed?
Another thing stakeholders should be on the lookout for when searching for an effective digital healthcare product is the type of trials that were employed when the research was conducted. Although Randomized Controlled Trials (RCTs) can be expensive and time-consuming, they remain the gold standard in proving effectiveness for clinical and medical interventions. If a trial was an RCT, the title of the trial would usually explicitly include ‘randomized control trial’ — as it’s something that companies would be keen to promote. As such there would typically also be information about the RCT on the company website.
The randomization procedures entailed in RCTs allow for the minimization of bias and, thus, the detection of valid and reliable intervention effects. This is because the randomization process balances both known and unknown participant characteristics across the intervention and control groups, allowing any differences in outcomes to be attributed directly to the intervention under scrutiny, as opposed to any epiphenomena or placebo effects. This is not possible with any other study design.
When designing an RCT, researchers must carefully select the target population, the interventions to be compared, and the outcomes of interest. Once these are defined, the number of participants required to reliably detect if an intervention effect exists should be calculated (i.e., a “power calculation”). Participants should then be recruited and randomly allocated to either the intervention or the comparator condition. Suitable comparator conditions may be passive control groups receiving no treatment or active comparators receiving treatment-as-usual, a placebo intervention, or an alternative intervention. It is important to ensure that at the time of recruitment there is no knowledge of which group the participant will be allocated to; this is known as concealment and is often ensured by using automated randomization procedures (e.g., computer generation).
3. Were the trial participants appropriately represented?
When looking into the trials, in general, ‘representativeness’ is most effectively achieved by randomly selecting an adequately large sample of participants from the defined target population; and in the case of clinical trials, by randomizing the allocation of participants into comparison groups. Beyond those key considerations, the representativeness of study samples should be critically appraised by considering the following three additional bullet points for consideration:
– Defining appropriate inclusion and exclusion criteria. However, it is not uncommon to see companies using strict inclusion and exclusion criteria in their publications in a manner that serves to increase the likelihood that the study sample will achieve positive outcomes. For example, selecting only users who are motivated (e.g., paid subscribers), those committing to using the intervention for a minimum period, and only including individuals with baseline severity levels, etc.
Imposing such strict criteria can bias the data and greatly reduce the generalizability of the study findings to real-world settings. Yet, the specifically tailored sample upon which the findings are based is typically not mentioned when companies make claims about the ‘evidence’ base underpinning their intervention. In most instances, it is good practice to define eligibility criteria as broadly as possible so that the findings are relevant to the full spectrum of people to who the intervention will be provided. Essentially, the key point here is that stakeholders should be wary that inclusion and exclusion criteria can limit the generalizability of findings.
– Verify that a representative study sample remains representative throughout the duration of the study. While some degree of attrition (i.e., dropout, loss to follow-up, or exclusion of participants) is largely inevitable, excessive attrition poses a threat to the internal and external validity of the findings. For instance, those who drop out of the study or who are lost to follow-up may be systematically different from those who remain in the study. Thus, simply ignoring everyone who did not complete a trial can bias results, usually in a direction that leads to an overestimation of the effectiveness of the intervention. It is, therefore, best practice to analyze the results of comparative studies on an ‘intention-to-treat’ basis as opposed to ‘per protocol’ analyses.
This means that all data on participants originally allocated to the intervention arm of the study, irrespective of whether they dropped out, should be analyzed along with the data on the participants who followed the protocol through to the end of the study. It is also important to pay attention to whether and how filters are used at every stage of analysis. Stratifying or filtering the data can be important to understand how outcomes vary in different populations, service types, and geographic locations. But it is not uncommon to see companies/ study authors applying specific filters (e.g., baseline severity thresholds) to the data to report on a particular subset of participants who showed greater benefit from the intervention relative to the sample.
The associated statistics are all too often the ones that companies proceed to proclaim on their website without clarifying that the evidence they have for those benefits is only based on a very specific subsample. The key point here is that stakeholders should be wary that the representativeness of study samples can change during the study and at the statistical analysis stage.
– Question the external validity of studies wherein participants were provided with a new smartphone, were paid to participate or were offered extra therapy services while using the DMHI. While such incentives may not necessarily invalidate the study itself, they may cast doubt on the applicability of the findings to individuals who do not receive the same incentives. More broadly, these concerns speak to the importance of examining whether DMHIs are likely to remain effective outside the controlled settings of a research trial, as a trial setting may give participants added motivation to engage with the program which might not be present in real-world settings. The key point here is that stakeholders should be wary that the provision of incentives to participate can bias the study findings – and lead to participants not being representative of the real-world use case of the product.
In short, there are several factors that can limit the extent to which the study sample is representative of the spectrum of people to whom the intervention is being marketed in real-life. Stakeholders should be aware that the conclusions drawn from a study regarding effectiveness, or the relative benefits/risks of a given intervention may not be applicable to the target population that the conclusions are being extended.
4. Has the research been published in a peer-reviewed journal?
Stakeholders should primarily be wary of companies that rely on blog articles and white papers that supposedly support the effectiveness of their DMHI. These self-publications point to “internal experiments” that endorse the product’s benefits but fail to provide adequate information for stakeholders to determine whether the results from these internal experiments are verifiable or reliable. Self-publication of articles as ‘preprints’ prior to formal review has become a popular means of scientific communication in many fields. While the value of using preprint servers (e.g., bioRxiv) to obtain valuable feedback on papers in advance of formal peer-review is well-recognized, stakeholders should be aware that approximately one-third of preprints posted on these servers never get published in peer-reviewed journals.
So-called predatory journals from unethical publishers are also increasing at an alarming rate. These journals offer opportunities for quick and easy publication which depends solely on payment and not formal peer review. While it can be acknowledged that publishing research of high academic standards is clearly not the primary mission of digital health companies when their products are destined to affect the health and well-being of people, holding them to a minimal standard of evaluation from the scientific community is essential. Also, participation in peer review is the best way we have to uphold this standard. As a desired minimal threshold, stakeholders should seek to establish that evidence of effectiveness has been published in a reputable peer-reviewed journal.
5. Has there been an appropriate level of transparency about how outcome variables are defined?
Lastly, stakeholders should seek to verify that a clear definition of outcome variables, or variables that are measured or examined to determine the effect of the intervention, have been provided when evaluating the evidence base of a digital healthcare product. Words like ‘engagement, ‘reduction’ and ‘improvement’ to describe outcomes are often used without adequate explanation about how these variables are defined or operationalized by a given company. Without clear definitions, an appreciation of the limitations of a study’s methods is also elusive. More generally, the pervasive use of inconsistent criteria to measure the same phenomena makes interpretation and comparison of results across companies and research studies very challenging.
Ideally, companies should be adhering to published reporting criteria for indices such as ‘recovery,’ and ‘reliable improvement’ when describing the effects of DMHI, and the associated sources for the definitions and criteria should also be cited. Resolving this issue will require a standardization of terminology across the industry. But, for the time being, it is important to generate awareness about how pervasive this issue is, and every effort should be made to determine whether reported outcome variables constitute meaningful constructs. For instance, although a one-point drop in scores on a questionnaire might be statistically significant, it may not be clinically meaningful or have any real-world relevance. Stakeholders should seek to verify that clear definitions of outcome measures, as well as their respective sources, have been provided, and should question the real-world value of the findings in instances where they have not.
These five questions and examples are just some of the many reasons why it is important to critically evaluate the quality, relevance, and generalizability of research before deciding how much credence to give to any reported findings or claims of DMHI. These examples also highlight how when published evidence is available, it can often be misrepresented in a manner that does not reflect the true conclusions that can be drawn from the published work. Therefore, we urge stakeholders to not only be satisfied with the mere presence of evidence but rather be informed of the need to interrogate both the quality of the evidence and the accuracy of the corresponding marketing claims subsequently made about the product.
Increased awareness of these pervasive issues of bias and misleading claims should empower stakeholders to make more informed decisions when evaluating and investing in DMHI. The hope is that this increased awareness will also, in turn, put pressure on the DMHI industry at large to improve standards of integrity, transparency, and fidelity to the truth, when reporting DMHI outcomes.
About Siobhán Harty
Siobhán is a Digital Health Scientist at SilverCloud (part of Amwell). She has a Ph.D. in Psychology, a Postgraduate Diploma in Statistics, and a number of additional years of experience in academia as a postdoctoral researcher at Oxford University and Trinity College Dublin. During her time in academia, she acquired expertise in a wide range of sophisticated methodologies spanning the fields of psychology, neuropsychology, and cognitive neuroscience. At SilverCloud, she is particularly passionate about optimizing experimental design and data quality, as well as improving our understanding of the factors underpinning inter-individual differences in responsiveness to our CBT programs.
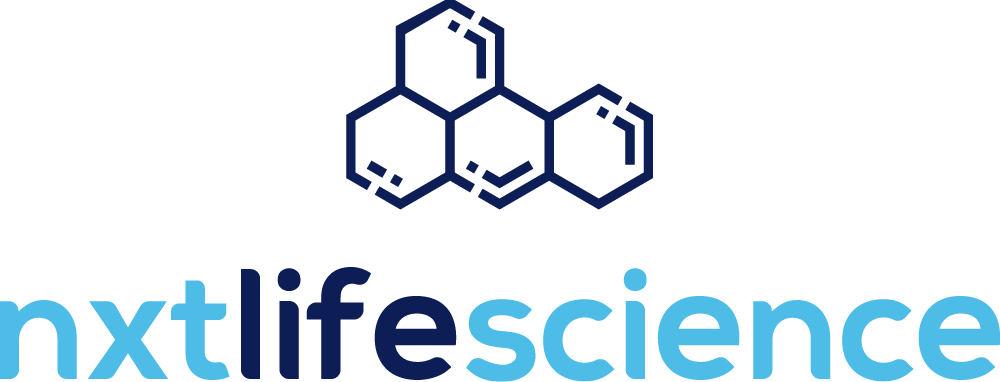
Keep it Short
By KIM BELLARD OK, I admit it: I’m on Facebook. I still use Twitter – whoops, I mean X. I have an Instagram account but don’t think I’ve ever posted….
Asian Fund for Cancer Research announces Degron Therapeutics as the 2023 BRACE Award Venture Competition Winner
The Asian Fund for Cancer Research (AFCR) is pleased to announce that Degron Therapeutics was selected as the winner of the 2023 BRACE Award Venture Competition….
Seattle startup Olamedi building platform to automate health clinic communications
A new Seattle startup led by co-founders with experience in health tech is aiming to automate communication processes for healthcare clinics with its software…