Digital Health
Medical Natural Language Processing Tech Has Come of Age
Dr. Tim O’Connell, Founder & CEO of emtelligent For many years, natural language processing (NLP) has held the promise of dramatically increasing…
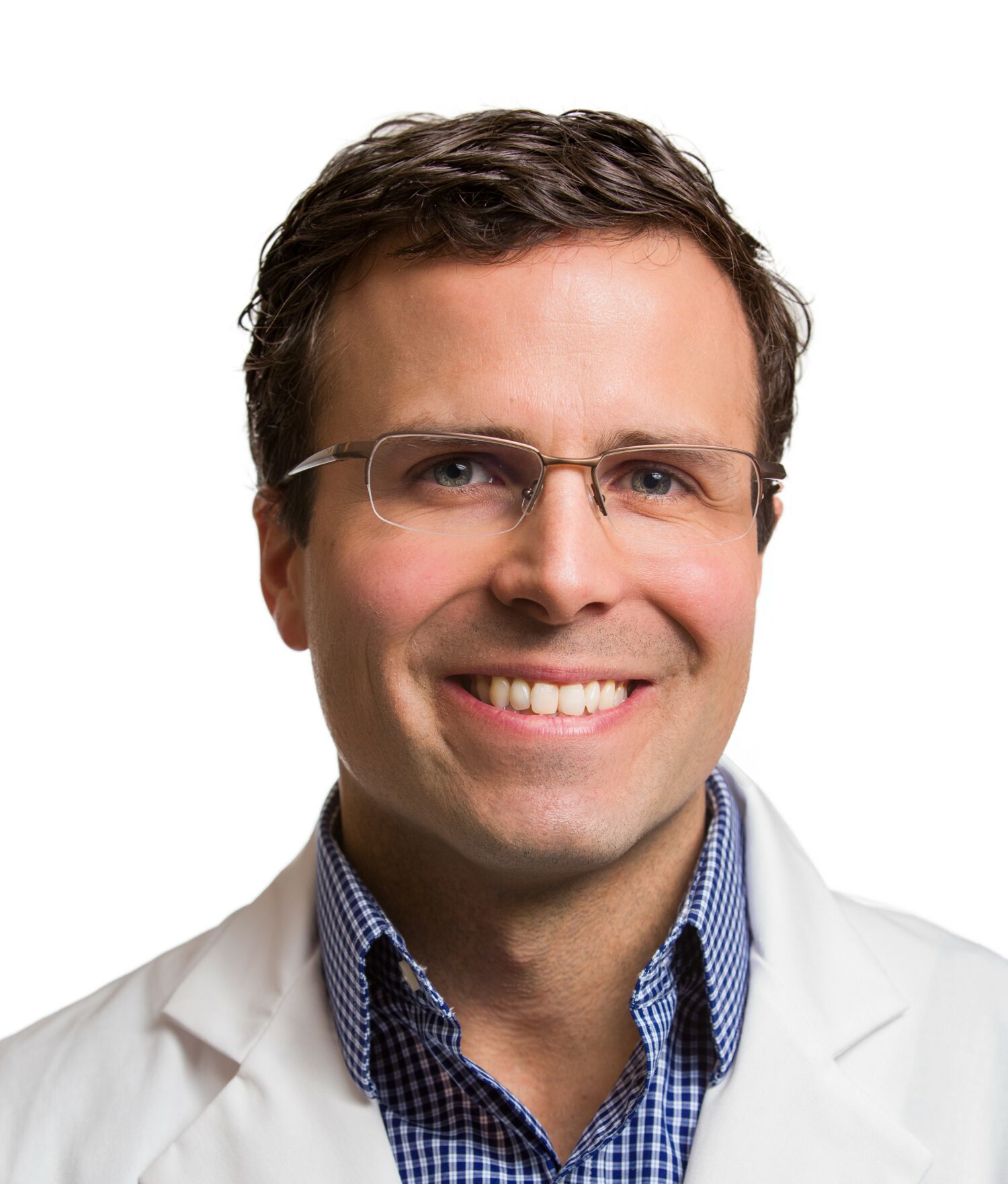

For many years, natural language processing (NLP) has held the promise of dramatically increasing the ability of healthcare organizations to quickly and accurately understand unstructured medical text in clinical notes. Using medical NLP, healthcare providers, clinical researchers, and payers would uncover meaningful insights hidden in unstructured text faster, with fewer errors, and at less cost than manual data review and analysis. This high-quality medical-grade data in turn would drive advances in understanding disease progression, assessing treatment efficacy, and detecting population health trends and other use cases.
Things haven’t quite worked out that way. While single-institution, single-document-type NLP projects have proven viable, dealing with the complexity of language across multiple institutions and document types has eluded accurate NLP.
One mistake healthcare organizations commonly make is they assume the medical NLP software they purchased is adequate for their use case. Yet these tools simply are not accurate enough to provide clinical-quality NLP because they are not fluent in the language of medicine. Medical vernacular is full of inherent complexities such as significant ambiguity, a special lexicon, and heavy use of localized medical shorthand. Add in the diversity of medical specialties and dearth of standards for the structure of medical documents, and it is clear that healthcare organizations require highly specialized medical NLP that leverages advanced technologies such as artificial intelligence (AI) and deep learning.
Fortunately, AI models have improved drastically with the advent of deep learning. Still, without any sort of medical expertise guiding the development of these deep learning models, users end up with results that basically say, “We found a whole bunch of things. Now you go figure out what is important.” That’s not exactly clinical-grade information.
What’s needed for a quality medical NLP platform is a combination of technology and medical expertise. By infusing deep learning models with specialized medical expertise, modern medical NLP software can help providers, payers, pharmaceutical companies, and clinical researchers get the most value from the data.
Limits of traditional NLP in medicine
While NLP undoubtedly has proved useful to researchers, the process involved can be labor-intensive and time-consuming. Let’s say researchers wanted to use NLP to find all patients in a target population who had appendicitis last month, with the data to be used in a white paper. Low-precision traditional NLP may identify 1,000 patients – but the NLP can be frequently wrong. As a result, a researcher must go through the data and confirm it all. Granted, that’s still better than the researcher laboring over manual chart reviews, but that still falls well short of an efficient and effective solution.
For other healthcare use cases – such as understanding human speech, computer-assisted coding (CAC), and clinical decision support – even “mostly accurate” software is nowhere close to acceptable. Healthcare organizations that implement a medical NLP platform that is extremely accurate will be able to apply their data to uses cases beyond research.
Choosing the right medical-grade NLP platform can be difficult for healthcare organizations that may not know precisely what features or functionality will work for them. Here are three things to look for in a clinical-grade NLP platform:
Accuracy
Does the platform provide enough accuracy for your organization’s purposes? For example, some platforms may have an algorithm for negation detection, the process of determining the presence or absence of conditions or diseases such as cancer or diabetes. However, the accuracy of these algorithms can vary depending on their ability to contextualize language in medical notes.
The ability of an NLP platform to accurately identify common medical terms, including slang, must be a priority to guarantee high levels of accuracy. Annotation software can perform the work of physicians who traditionally would annotate thousands of medical reports – and do it much faster – but the medical NLP solution must be able to keep pace in speed and accuracy.
Features
Healthcare organizations must know the specific functionalities of a medical NLP platform. Which ontologies does it support (SNOMED, RadLex, MEDCIN, ICD-10, etc.)? Does it identify questions or uncertainty? Can it extract insights from the unstructured text of clinical, diagnostic, and semi-structured reports?
Another important feature involves the platform’s ability to identify relations within data. Does it identify measurements? Or dates? If the platform is analyzing a report about a patient with a history of appendicitis, does the algorithm understand that appendicitis happened in the past? Or does it just say that the patient has appendicitis now? If the report contains a statement that the patient’s mother has breast cancer, does it attribute breast cancer to the patient, or can it accurately identify the experience?
Deployment location
Many medical NLP vendors offer only cloud-based services, but not all healthcare organizations are eager to send their patient data to the cloud. Today’s focus on information safety makes cloud-based solutions in this space less attractive. For those organizations, on-premises medical NLP platform deployments are essential.
Conclusion
Relative to the requirements of provider and payer organizations, medical NLP for too long has left much to be desired in accuracy and flexibility. Recent advances in AI now make it possible for medical NLP to help healthcare organizations leverage highly accurate data for clinical work, research and drug development. Healthcare organizations should ensure a medical NLP platform is accurate enough and includes the features they need to get the most from their data.
About Dr. Tim O’Connell
Dr. Tim O’Connell is the founder and CEO of emtelligent, a Vancouver-based medical NLP technology solution. He is also a practicing radiologist, and the vice-chair of clinical informatics at the University of British Columbia.
artificial intelligence
ai
software
healthcare
medicine
medical
pharmaceutical
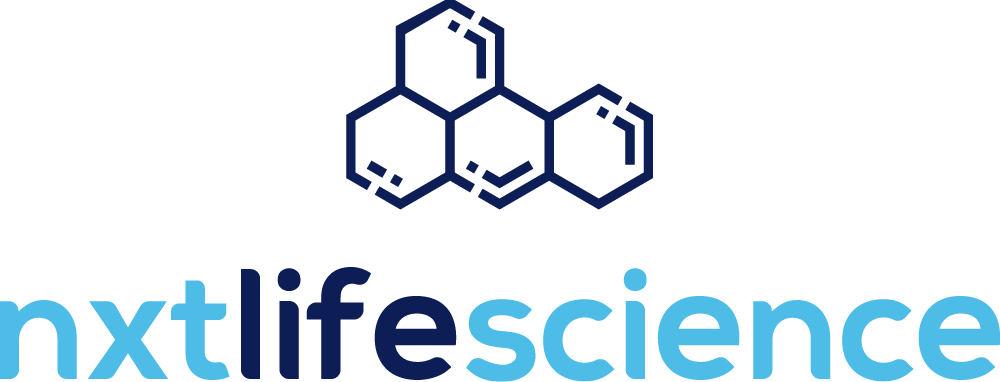
Keep it Short
By KIM BELLARD OK, I admit it: I’m on Facebook. I still use Twitter – whoops, I mean X. I have an Instagram account but don’t think I’ve ever posted….
Asian Fund for Cancer Research announces Degron Therapeutics as the 2023 BRACE Award Venture Competition Winner
The Asian Fund for Cancer Research (AFCR) is pleased to announce that Degron Therapeutics was selected as the winner of the 2023 BRACE Award Venture Competition….
Seattle startup Olamedi building platform to automate health clinic communications
A new Seattle startup led by co-founders with experience in health tech is aiming to automate communication processes for healthcare clinics with its software…