Digital Health
Why Researchers Should Explore New Apps of Machine Learning
Michael Munsell, Director of Research at Panalgo As the amount of real-world data (RWD) in the pharmaceutical industry continues to grow, so does the usage…


As the amount of real-world data (RWD) in the pharmaceutical industry continues to grow, so does the usage of machine learning (ML) to analyze that data and gain insights. In fact, in a recent survey, 95% of life sciences executives said they expect to utilize ML in the next few years to generate real-world evidence (RWE) from this data.
With such a large and growing volume of data available, researchers may be wondering how they can take advantage of all of it to enhance and expand the impact of their research. Many organizations have already adopted ML for certain uses, but in order to maximize the value of data, researchers will need to move beyond the most common applications of the tool. By leveraging ML in new and innovative ways, organizations can push the boundaries of their research and uncover insights that will truly impact patient lives.
How machine learning is currently being used
Currently, the most common use of ML involves predictive modeling for high-stakes scenarios. Researchers input RWD into a model, and the algorithms predict outcomes based on that data. These predictions can be used as RWE to incorporate into regulatory submissions or to inform further research, or they can be leveraged to guide decisions and take further action.
One example of the former use case is utilizing ML to understand optimal treatments for certain diseases. For example, for patients with metastatic breast cancer (MBC), ML models can analyze information including age, date of diagnosis, cancer stage, and other factors to predict the treatment regimens that will maximize overall survival and speed up time-to-treatment discontinuation. This insight could be used to inform treatment choices and potentially improve outcomes for patients with MBC, once clinically validated.
In the latter case, predictive modeling can have tangible benefits if the findings are actually used to make decisions around patient treatment. Predictive modeling has been particularly successful in assessing sepsis outcomes and determining treatment timelines, for example. Researchers have used ML to predict mortality over time for patients with sepsis and then, using this insight, decided at which point to administer drugs to patients. Using this approach, researchers have successfully been able to reduce mortality for sepsis patients.
Where researchers can expand their uses of machine learning
Researchers have proven the benefits of utilizing ML to make predictions, but now we need to leverage the technology even further. In order to maximize the potential of the technology for impacting patient lives, researchers must understand not just what the predicted outcomes are, but why they are happening. Causal inference is a well-defined concept in statistics, but what’s new is using ML to derive causal inference. Predictions from ML models can be highly valuable, but if we cannot explain them, then the picture is incomplete. Causal inference can help validate ML outputs by providing explanations for the insights that researchers are finding, and this is an application of ML that researchers should pursue.
Researchers should also further explore the applications of unsupervised machine learning. Unsupervised ML involves analyzing and finding patterns within datasets without any reference to known outcomes. While ML typically involves predicting future outcomes based on known data of past outcomes, unsupervised ML leverages data that is not yet understood to discover hidden patterns and insights in the underlying structure of the data. Predictive ML is useful for answering specific questions, but unsupervised ML can allow researchers to explore questions they hadn’t even thought of, generating hypotheses and truly novel insights.
One specific application of unsupervised ML is identifying and understanding patient subgroups, such as the subset of Alzheimer’s patients with certain characteristics. In one recent study utilizing unsupervised ML, researchers found that female Alzheimer’s patients with a younger age of disease onset, as well as comorbid depression and anxiety, were more likely to have quicker rates of disease progression and worse outcomes. When researchers can define such subgroups, they can focus their attention on further investigating these groups and uncover the best methods to potentially improve outcomes.
Best practices for applying machine learning
Just because ML is being used does not mean it’s being used correctly. We’ve seen great progress in ML becoming more accessible and widely utilized, but researchers must ensure that they’re using the tool appropriately. For those incorporating ML into their studies, documentation and transparency are key, especially when it comes to regulatory submissions.
While regulatory institutions such as the FDA used to be focused simply on the findings and insights within a regulatory submission, there has been a shift towards greater attention to the underlying models and data used to conduct the research and uncover those insights. To build a successful submission, researchers must meticulously document all parts of their investigations. As a rule of thumb, methods and findings should be understandable to someone who was not directly involved in the research.
Researchers may choose to utilize software that automatically documents and creates a report of all methods and materials, including the raw data, models used to analyze the data, and outputs from the analysis. However, regardless of whether the documentation is done automatically or manually, studies must be fully transparent, understandable, and reproducible. This will help ensure that researchers not only have successful submissions but that their research truly has a noticeable reach and impact.
The future of machine learning in life sciences
When ML first came onto the life sciences scene, many people were skeptical about whether the tool was actually valuable or if it was overhyped. However, as it has become more widely utilized, researchers have increasingly found its capabilities useful, particularly when it comes to handling high-dimensional healthcare data. And, thanks to recent guidelines from the FDA around using RWD and RWE in regulatory submissions, comfort with using ML to analyze RWD continues to grow.
We’re not yet at the point where all researchers, regulatory institutions, or patients fully understand ML, but we are seeing a shift toward embracing the technology more. Researchers have realized the benefits of ML in augmenting traditional analytical methods, reducing biases, and strengthening results. For the FDA, providing guidelines around RWD usage and beginning to acknowledge RWE steps in the right direction. The next step will be the FDA fully accepting the use of ML to generate that RWE and its insights.
Now that we’re more comfortable with the basic usages of ML, exploring new applications of it – while ensuring that it’s being used appropriately – is the next frontier. As researchers continue to realize the potential of ML for impacting patient lives, expanded usage is on the horizon.
About Michael Munsell
Michael Munsell, PhD, is the Director of Research at Panalgo, where he is responsible for managing the internal and collaborative research agenda as well as contributing to the scientific development of the IHD platform, including prototyping and validating new machine learning models for IHD Data Science. Mike has a wealth of experience in RWD study design and has authored several publications in a variety of fields, including health economics, outcomes research and data science. He holds a PhD from Brandeis University, with a focus on computational economics, and an undergraduate degree in Economics from the University of Michigan.
machine learning
apps
software
healthcare
pharmaceutical
fda
research
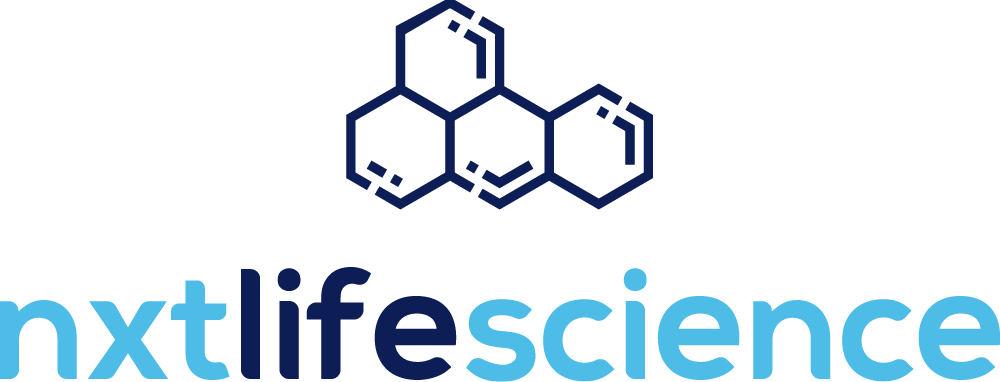
Keep it Short
By KIM BELLARD OK, I admit it: I’m on Facebook. I still use Twitter – whoops, I mean X. I have an Instagram account but don’t think I’ve ever posted….
Asian Fund for Cancer Research announces Degron Therapeutics as the 2023 BRACE Award Venture Competition Winner
The Asian Fund for Cancer Research (AFCR) is pleased to announce that Degron Therapeutics was selected as the winner of the 2023 BRACE Award Venture Competition….
Seattle startup Olamedi building platform to automate health clinic communications
A new Seattle startup led by co-founders with experience in health tech is aiming to automate communication processes for healthcare clinics with its software…