Medtech
A matter of trust
AI technologies have the capacity to revolutionize the practice of medicine – if human HCPs can learn to trust them first.
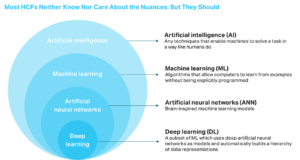
A matter of trust
By Jonathan Wert, Kathy Belk, and Jan-Willem van Doorn
This is the second in a series of three articles by HCG thought leaders that will be appearing in Med Ad News exploring the impact and application of artificial intelligence in a number of areas touching healthcare and healthcare communications. Part one discussed the next generation of lit reviews. The final article, discussing AI and creative development, will appear in June.
Ever wonder how much artificial intelligence (AI) has impacted the actual “bedside” practice of medicine?
Practically speaking, on the scale of what might be possible, not as much as you might think. If we take as the beginning of the continuum the release of PONG and the end as Robert Picardo’s Emergency Medical Hologram in Star Trek Voyager, a fully virtual being actively and autonomously practicing medicine, well, let’s just say there’s a lot more path in front of us than behind us.
That said, though, the use of AI and its related technologies has been creeping into clinical practice in a variety of quiet but significant ways. We’ve encountered clinical deployments of AI in logistics, a la scheduling, triage, and ordering of supplies and vaccines; in disease prediction and diagnosis, and even in calculating treatment effectiveness and predicting outcomes. Certain subspecialties like radiology, cardiology, and oncology may have found themselves more at the vanguard of AI use in clinical practice by their very nature as vast consumers and analysts of unstructured data. Just as human reviewers get fatigued working through thousands of articles for a literature review (see our article on AI and lit reviews in the December issue of Med Ad News), radiologists have to eyeball MRIs, CTs, ultrasounds, and radiographs, each basically a vast amount of unstructured data – and a place where an untiring AI with the ability to seek out patterns and deviations has the potential to add efficiency to the process. In fact, FDA has approved or cleared for use at least 343 AI- or machine learning-enabled devices in the past 25 years, the large majority of them since 2016, and seven out of 10 in radiology at the time of this publication.
Before descending too much further into this particular rabbit hole, we should pause for a moment to define our terms. In the broader discussion of the impact of advanced AI-related technologies in our industry, many have often been incorrectly using interchangeable terminology, often with little regard for its actual meaning, and your typical HCP or brand manager could be forgiven for not understanding all the nuances. We’ve included the illustration on the right for an overview of the terminology.
So what do the current implementations of all this in clinical practice actually look like? One company is using AI and deep learning to improve the quality of MRI images. Another is deploying algorithms to detect and distinguish medical conditions in the paranasal sinus, identify brain tumors, and predict patient outcomes and levels of surgical risk. One deployed AI-based deep learning atrial fibrillation risk prediction model was trained using 100,000 ECGs from more than 40,000 patients; it can explicitly predict time to AF using 12-lead ECG data. We also have seen an FDA-authorized autonomous AI diagnostic system for detecting diabetic retinopathy based on digital images uploaded to a cloud server by a non-specialist HCP. AI models have reduced the false positive rates in mammogram screenings by 69 percent; artificial neural networks are able to predict breast cancer-related survival rates and the risk of colorectal cancer. These technologies are out there, actual HCPs are using them, and they are growing smarter and more accurate every day.
But. But the reality is that few commercial AI-based applications, even in radiology, have actually been implemented on a large scale by health systems.
Why not? Because the use of AI and its relatives in clinical practice applications touches on some sensitivities that use elsewhere does not, or does to a lesser degree.
Near the top of the list is responsibility. Who is responsible for the algorithm’s decisions? Is it the developer? The HCP? The hospital? Put another way, if Dr. AI makes a mistake and a patient is injured in some way because of it, who does the patient sue?
Next is evidence. While many AI-based clinical tools claim to be based on large samples of data, very few of them have actually faced the music of a large-scale third-party published clinical study as pharmaceutical drugs and devices have. And HCPs, a naturally questioning lot, may be wondering where all that data came from, who selected it, and why. After all, any AI tool is only as smart and unbiased as the people and data that are used to train it – and anyone who’s been reading the data science news lately is surely aware that AI algorithms can be just as prone to bias as humans are. And even when the source data to create an algorithm is robust, AI-based clinical tools often rely on real-time data input and require the input data to be clean and standardized.
Then there’s data ownership. Once an AI-based application is actually implemented in a clinical setting, it needs to use the real patient data it would be encountering in order to learn and improve – the essence of machine learning. But who owns that data? Will patients accept the idea of this modern-day HAL (the quasi-sentient AI computer in 2001: A Space Odyssey) using their experience to its benefit? Will HCPs? If not, the application would become the equivalent of a doctor who stops studying doctoring as soon as he graduates from medical school and never learns anything new; it would never get any better or more efficient than it is on day one.
Another challenge is the integration of AI tools into actual practice workflow. We’ve seen some of them find their way into EMRs, but if they aren’t built into workflow, associated with an alert, protocol, or order, and integrated in a way that actually reduces workload for HCPs, their use doesn’t become widespread. And of course the resources available to implement these types of tools are limited, no matter what the size of the practice might be.
An additional challenge is plain old economic incentive. As Ajay Agrawal, Joshua Gans, and Avi Goldfarb write in Power and Prediction: The Disruptive Economics of Artificial Intelligence, “If we simply drop new AI technologies into the existing health-care system, doctors may not have the incentive to use them, depending on whether they will increase or decrease their compensation, which is driven by fee-for-service or volume-based reimbursement.”
Lurking underneath many of these sensitivities is a basic question of trust. To a typical HCP, an AI platform reading MRIs looks an awful lot like a black box. One can easily imagine their thoughts; “I spent eight extra years in school to learn how to read and interpret those things, it’s really more art than science, and now I’m supposed to trust this oversized doorstop to do it for me?” HCPs can ask other HCPs where they went to med school, where they did their residency and fellowship, where they’ve practiced, what research they’ve done, what experiences they’ve had, what brought them to a place where their opinion is what it is. It’s not quite as easy to ask an AI those same questions.
Larger minds than ours are already attempting to work out pathways around the trust problem in clinical applications of AI. In “Next-generation artificial intelligence for diagnosis: From predicting diagnostic labels to ‘wayfinding,’” an article that appeared in JAMA in December 2021, the authors advocate for a shift in the use of AI in a clinical context, from simply making diagnoses based on data input to supporting the HCP and patient along the diagnostic pathway. “A new generation of AI is needed,” they argue, “that considers the dynamism of the diagnostic process and answers the questions of where are the clinician and the patient on the diagnostic pathway and what should be done next.” Tools of this new generation would help HCPs understand where they are on the diagnostic pathway and help them choose the best path forward and reduce uncertainty, but the HCPs would continue to independently analyze data and make final decisions.
Whether such an envisioned new generation comes to pass or not, it’s clear that AI offers the opportunity to transform clinical practice for the better. We don’t believe that AI will ever replace human HCPs, the Star Trek Voyager Emergency Medical Hologram notwithstanding. Too many parts of the healthcare continuum still require distinctly human characteristics like empathy, the capacity to comprehend and respond to unpredictable or nonlinear events, outside-the-box thinking. But HCPs that understand and use AI platforms will absolutely be better practitioners than those that do not. So we as brand managers and communicators need to help our clients and our HCP audiences to embrace and facilitate adoption of AI and machine and deep learning in clinical practice.
How? Baby steps. When encountering something foreign, hard to understand, potentially threatening, trust is built slowly. One possibility is to encourage a parallel process a la our example in the previous article about literature reviews; i.e., one HCP, one AI, reviewing and comparing results, with the HCP having the final word. Over time the AI improves, perhaps sees things the HCP doesn’t, and the HCP gains trust. Another is to start publishing AI algorithms, methodologies, and data sources in the medical literature. All sorts of other tools that HCPs use have established their credibility through study and peer review in medical literature; this one, with its extraordinary potential to impact the diagnostic process as few other tools have, ought to establish its bona fides similarly.
But more importantly than what happens when HCPs start working with AI is what happens before. Are the developers of the AI platform engaging with its potential users before they become users? Are they offering transparency into where the underlying data came from and why it was chosen? Are they presenting a balanced view of the ethical challenges that users might face and empaneling ethics review boards to explore those challenges? Are they showing how patient data can be protected while still maintaining the learning capabilities of the platform? Are they seeking out and attacking potential biases – and explaining to their potential users how they are doing so? The way to build HCP trust in AI platforms is to slowly and surely drain the mystery out of them, and the way to do that is through transparency, collaboration, and communication.
Are you or your company considering or developing or implementing an AI project for clinical practice? Let’s talk about how we can help educate HCPs and move them along the adoption spectrum from skeptical to advocacy.
Jonathan Wert, M.D., is senior VP, clinical strategy; Kathy Belk is VP, strategic operations and innovation; and Jan-Willem van Doorn, M.D., Ph.D., is chief transformation officer, Healthcare Consultancy Group.
vaccines
medical
healthcare
diagnostic
radiology
medicine
health
hospital
pharmaceutical
devices
artificial intelligence
ai
emrs
machine learning
fda
research
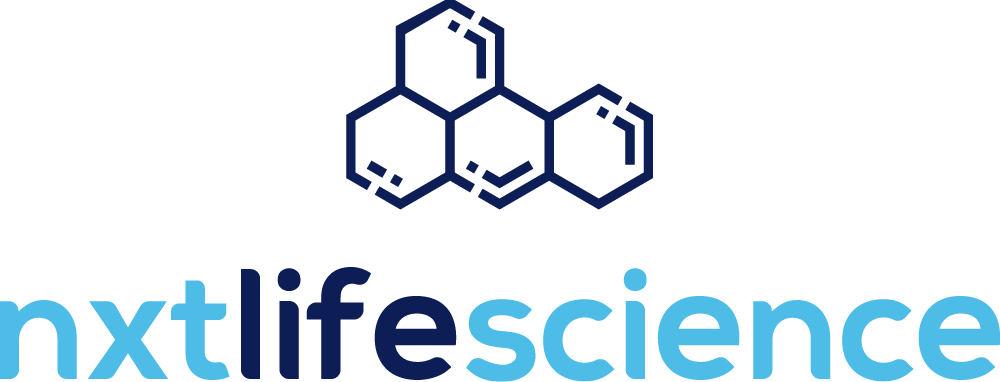
ETF Talk: AI is ‘Big Generator’
Second nature comes alive Even if you close your eyes We exist through this strange device — Yes, “Big Generator” Artificial intelligence (AI) has…
Apple gets an appeals court win for its Apple Watch
Apple has at least a couple more weeks before it has to worry about another sales ban.
Federal court blocks ban on Apple Watches after Apple appeal
A federal appeals court has temporarily blocked a sweeping import ban on Apple’s latest smartwatches while the patent dispute winds its way through…