Life Sciences
AiThority Interview with Gideon Lapidoth, PhD, CEO of Enzymit
The post AiThority Interview with Gideon Lapidoth, PhD, CEO of Enzymit appeared first on AiThority.


Please tell us a little bit about your role and responsibilities at Enzymit.
As CEO and Co-founder of Enzymit, in addition to “traditional” startup CEO roles, such as management, strategy, funding, and business development, I am very much involved in technology development.
Given my background in computational protein design, I have domain expertise in both the algorithmic and biochemical aspects of our operations. That means that I know what we should be working on (i.e. which enzyme we should be developing or those chemical reactions that are of particular interest) and also how we should be getting there (which algorithms or models could potentially get us the desired results). This is a fantastic place to be in, since it enables us to see solutions to problems that other biotech companies might not be aware of, as the technology to build these enzymes is so novel.
Could you tell us more about the ideal customer profile of Enzymit? Which industries rely on your solutions and services?
Our vision is to disrupt the traditional chemical industry. Using our cell-free platform, we can produce anything from polymers and pharmaceuticals to food and beverage supplements. Within this enormous opportunity space we have zeroed in on the specialty chemicals sector. These molecules differ from commodity chemicals in that they are sold based on their function or performance, rather than their composition and are either too complex to produce using traditional chemistry or too expensive to produce using fermentation based bio-production.
Our technological advantage gives us a unique positioning, enabling us to manufacture complex molecules while remaining cost competitive. This is thanks to our focus on cell-free based production, rather than organism-based bio-production, which has dominated the field over the past decades.
We have already developed a novel enzymatic process for manufacturing hyaluronic acid, a crucial component in the cosmetic industry.
Similarly we have developed an enzymatic process for manufacturing prebiotics only found in human breast milk. These prebiotics are crucial for infant development, yet they are currently absent from nearly all commercially available infant formula. We are also developing bio-based solutions for the defense industries. We are thus actively engaging with a variety of global manufacturers and providers across multiple industries who are looking for alternative solutions to optimize their production processes.
Tell us more about your AI capabilities and how these fit into your product development plans.
The founding team at Enzymit are veterans of the Rosetta community, which until recently was the most successful software suite ever developed for computational protein design and modeling. That is to say we have an extremely strong background and deep understanding in the specific problem domain of computational protein design. As new deep-learning architectures are introduced, we constantly evaluate and adapt them to our specific challenges.
From the start, we identified cell-free manufacturing as an approach that is ripe for disruption. Bio-production has been traditionally based on fermentation, utilizing whole organisms to manufacture novel materials.
However, the only parts of the organism that are essential for production are a narrow set of enzymes that perform the actual chemical catalysis. The rest of the machinery in the organism (more than 20,000 different enzymes) are dedicated to keeping it alive and thriving. Consequently, the effort, resources and energy that are required to both sustain those cells and generate commercially relevant amounts of material is a major disadvantage of this approach.
With cell free production, only the enzymes that actually take part in the synthesis of the desired compound are put in the reaction mix. Thus, the theoretical yield is close to 100%, as no energy flows towards cell maintenance. However, cell-free production has been considered extremely challenging, the main hurdle being the enzymes themselves and how to synthesize highly robust and functional enzymes cost-effectively to suit manufacturing needs. These challenges can be directly addressed using computational design tools.
For each of these challenges we have developed specific AI-based tools.
For example, for the challenge of enzyme stabilization in harsh extracellular environments we built a model that combines large language models (LLM’s) with graph neural networks (GNNs). Each architecture reflects a different characteristic of proteins. LLMs have been successfully used in protein design, given that proteins are essentially a language where each amino acid comes together to form the protein “sentence.” GNN’s enable us to encode the three-dimensional attributes of the protein. Using the two architectures, our model ThermIT can take as input any protein sequence and introduce dozens of amino acid changes (mutations) that increase the overall thermal resistance of the protein without negatively affecting its activity.
To address the challenge of novel enzyme activity we developed an approach that relies on the modification of enzymes’ three-dimensional shapes. An enzyme’s function (or any protein for that matter) is directly tied to its shape. However, designing novel protein shapes is one of the toughest challenges in computer science. We developed the CoSaNN algorithm to specifically address this. This allows us to sample new protein conformations that are realistic and natural-like while at the same time vastly increasing our available conformation space.
We are currently working on a newer version of this algorithm that utilizes diffusion models to create novel conformations. The main motivation to use these models is the ability to fine-tune enzyme structures for specific molecules and to avoid using sampling which is more computationally intensive.
Could you tell us about CoSaNN and SolvIT?
How do these influence the ongoing research happening in the enzyme manufacturing industries?
CoSaNN and SolvIT were actually developed a short while after AlphaFold2 was released and have been a part of our computational production pipeline for over a year. Each algorithm was specifically developed to address a certain challenge we faced when it came to designing new enzymes for the manufacturing industries. SolvIT was built to improve the enzyme production yields in bacterial expression, which directly translate to cost of production. CoSaNN was developed to enable the design of enzymes with novel activities, which is vital for expanding the usability of enzymes across industries.
CoSaNN and SolvIT are good demonstrations of what we think practical protein design in commercial settings should look like – lean, targeted algorithms for specific challenges, rather than trying to build a catch-all solution.
The ideal protein design model would produce proteins tailored for specific functions when given a prompt that details its exact requirements (for example, “design an enzyme that can catalyze the conversion of CO2 to methane” or “design an antibody that binds the SARS-CoV-2 spike protein”). However, they currently lack the accuracy and reliability necessary for practical use in the industry. Failure in commercial projects is detrimental and so robustness in a variety of scenarios and use cases is vital.
As a startup, our goal is to develop swift and pragmatic solutions. Experience has taught us that domain expertise still plays a crucial role when it comes to building new algorithms to address the challenges of protein design. AI has still not reached a level of proficiency where generic prompts can produce the desired results. I would even venture to say that it is not enough to build “protein models” but that some of the models need to be fine-tuned to the specific protein family or function.
It is also clear to us that there is a finite set of rules governing protein structure and function. This is why computational protein design algorithms enjoyed some success, even before AI became ubiquitous. The significance of this is that we believe that there is enough extant data to train the next generation of AI-based protein design algorithms that will be able to solve the remaining challenges, such as one-shot novel catalyst design.
We are currently working on such tools while simultaneously maintaining and improving more ad-hoc algorithms.
What is your take on the future of AI models in biocatalysis?
There are two levels to this:
On the more practical application side, we believe that within the next two years we will see models able to solve nearly every existing protein design challenge and capable of generating novel biocatalysts on-demand with minimal effort from the user. This is almost on par with “AI singularity.”
There will be almost no industry on earth that would not be directly affected by this development. Just imagine cheap hydrogen production, direct conversion of CO2 to useful feedstocks, bioremediation, novel pharmaceuticals, cheaper food manufacturing, to name but a few. What is even more exciting is how accessible this technology can be – not controlled by highly skilled individuals or organizations but completely democratized. This brings us to the second level.
From its inception, AI brought about an open environment in which most developments are shared publicly. This has been the case for language models, vision, robotics, and more. As one Google engineer famously mentioned in a memo, “We have no moat.” What he meant was that Google as a company is threatened on the AI front, not by companies such as OpenAI, but by the open source community, which is faster, more adept and more innovative.
As an example, while Google and OpenAI focused on proprietary chat engines such as Bard and ChatGPT, and the resource-heavy language models that power them, Meta’s LLaMA was released as open source. Soon thereafter, the AI community adapted LLaMA to almost match the performance of ChatGPT and Bard at lower costs while optimizing it for devices, including laptops and phones, and improving training speed. We are also seeing this trend in protein design. Companies that previously marketed themselves as offering computational protein design tools and services have either pivoted to focus on specific products or otherwise gone extinct.
At Enzymit, we believe that the driving engine of innovation will be the open source community, which is spearheaded by groups such as the Baker Lab, the AlQuraishi Lab and others. Companies will continue to fine-tune these models for their own requirements and proprietary data sets. Meanwhile, biotech companies will find success by focusing on end products that provide real value.
Enzymit is steering towards real products, which, in contrast to AI models, are better protected and can serve as a competitive advantage in business.
Thank you, Gideon! That was fun and we hope to see you back on AiThority.com soon.
[To share your insights with us, please write to sghosh@martechseries.com]
Gideon Lapidoth is CEO and Co-founder of Enzymit, a bioproduction platform company developing cell-free enzymatic manufacturing technology. He is an expert in developing advanced computational algorithms for the design and creation of novel antibodies and enzymes with superior accuracy and activity.
Enzymit is building a cell-free production platform that will make bioproduction faster, simpler, cost-effective, and sustainable. The company leverages complex computational design and deep learning algorithms to create novel enzymes for use in real-world settings. These highly stable and robust enzymes can withstand higher temperatures and work for longer than ever before to enable production of novel molecules in a more efficient and environmentally friendly manner.
The post AiThority Interview with Gideon Lapidoth, PhD, CEO of Enzymit appeared first on AiThority.
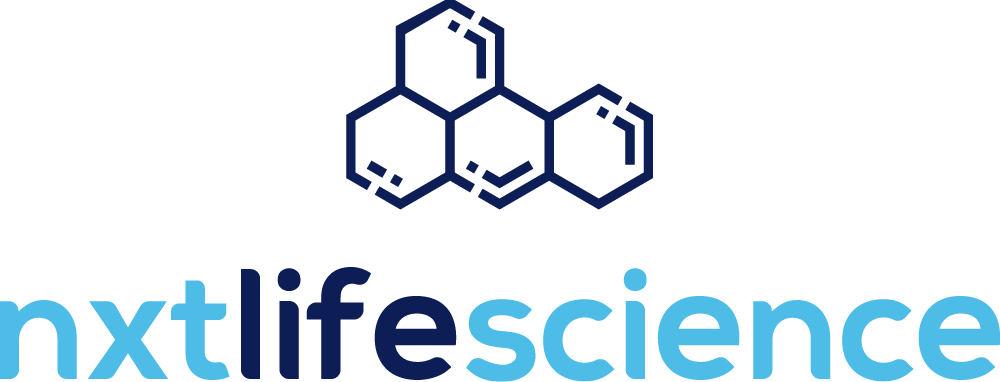
Wittiest stocks:: Avalo Therapeutics Inc (NASDAQ:AVTX 0.00%), Nokia Corp ADR (NYSE:NOK 0.90%)
There are two main reasons why moving averages are useful in forex trading: moving averages help traders define trend recognize changes in trend. Now well…
Spellbinding stocks: LumiraDx Limited (NASDAQ:LMDX 4.62%), Transocean Ltd (NYSE:RIG -2.67%)
There are two main reasons why moving averages are useful in forex trading: moving averages help traders define trend recognize changes in trend. Now well…
Asian Fund for Cancer Research announces Degron Therapeutics as the 2023 BRACE Award Venture Competition Winner
The Asian Fund for Cancer Research (AFCR) is pleased to announce that Degron Therapeutics was selected as the winner of the 2023 BRACE Award Venture Competition….