Life Sciences
Computational Tools Take Advantage of the Data Deluge
Instead of continuing to rely on trial-and-error testing, pharmaceutical scientists are increasingly turning to computer software to create better treatments.
The…

Besides developing safe and effective new drugs, the pharmaceutical industry is constantly engaged in two additional activities: improving production speeds and reducing costs. All of these activities can be streamlined through the application of advanced computational technology.

Optibrium
No approach to coming up with new drugs, however, can bypass the need to grapple with complexity. As Robert Abel, PhD, executive vice president and chief computational scientist at Schrödinger says, “Drug discovery is a massive multiparameter optimization challenge.” Nonetheless, it remains the case that this challenge is better assigned to a computational platform than to a researcher in a wet lab.
“Computational tools enable scientists to rigorously explore many optimization strategies and quickly target the most promising compounds based on many factors, including target activity and physicochemical and pharmacokinetic properties,” says Matthew Segall, PhD, CEO at Optibrium. “Such tools can dramatically cut the number of false leads pursued, experiments needed, and overall cost and timelines of the discovery process, while simultaneously uncovering more opportunities than could be investigated experimentally.”
Taking on the data challenge

BioSolveIT
Despite the obvious benefits of computational drug design, scientists face fundamental challenges in applying this approach. For example, “it has been a huge challenge to predict 3D protein conformations—what a protein really looks like in space,” says Marcus Gastreich, PhD, senior vice president of application science at BioSolveIT. “We’ve made a huge leap forward using technologies that rely on artificial intelligence, but everything comes with a price.” That price is huge amounts of data—almost too much data, which requires time and advanced algorithms to explore.
When helping a customer look for a potential new drug, Gastreich and his colleagues at BioSolveIT take on these challenges with a three-tiered approach. The first tier is the search through purchasable molecules. “We use our infiniSee platform to search through the zillions of molecules out there,” Gastreich asserts. “That was impossible a few years ago.” The platform uses algorithms that run on standard computer hardware but can search through trillions of chemicals within minutes. The results are available for purchase directly from BioSolveIT’s partners such as Enamine, WuXi AppTec, and eMolecules.
The second tier is the examination of selected chemicals in three dimensions. As Gastreich says, “We give customers meaningful visualizations so that they can make informed decisions.”
The third tier is combinatorial optimization. At this tier, a wide range of structural variations can be evaluated for their ability to fit to target sites that are involved in specific disease pathways. Overall, BioSolveIT provides substructure searches, similarity screening, and scaffold hopping in trillions of molecules, all of which run on standard hardware.
In the future, the data challenge will grow. “Chemical space is estimated to be on the order of 1060 molecules,” Gastreich says. “So, we should be ready to cope with even larger sets of data and have a comprehensive toolset that allows navigation in this huge amount of data.”

Increasing speed and accuracy
The growing body of data involved in computational drug design also impacts two ongoing issues in this field. “Historically, speed and accuracy limited the impact of computational workflows to drive drug design because they were too slow to be incorporated into experimental design cycles,” Schrödinger’s Abel explains. “They offered poor predictive value. So, they were viewed as ancillary tools with limited impact in the design process by many drug hunters.”
In an effort to improve the speed and accuracy of computational drug design, scientists at Schrödinger invested 30 years in research and development. According to Abel, this commitment enabled the company to build “a platform that combines the accuracy of physics-based methods that can predict key properties—such as potency, selectivity, solubility, permeability, as well as ADMET [absorption, distribution, metabolism, excretion, toxicity] properties—with the speed and scalability of machine learning and artificial intelligence.”
Making the most of such methods, however, also depends on the hardware. This point is emphasized by Abel, who notes that the Schrödinger “platform has been optimized to run on high-performance GPUs—graphic processing units—both on the cloud and on high-performance computing hardware, which dramatically accelerates the speed of calculations.”
Abel notes that Schrödinger licenses its “integrated in silico molecular discovery and design platform to pharmaceutical, biotechnology, and academic researchers across the globe.” This platform includes two fundamental tools: Maestro and LiveDesign.
“Maestro is our 3D molecular modeling environment where users can build and run simulations,” Abel says. “These include docking, quantum mechanics calculations, molecular-dynamics simulations, free energy calculations, and more.” He adds that Maestro can connect with LiveDesign, which is Schrödinger’s collaborative enterprise informatics platform.

“LiveDesign provides centralized access to virtual ideas, wet-lab project data, and powerful computational predictions to the entire project team, including nonexperts,” Abel notes. “This allows discovery teams to crowdsource ideation, digitally test design ideas, and actively learn from the data to iteratively revise strategies and drive more productive design-make-test-analyze cycles.”
Creating communities
Making the tools of computational drug design available to a wide range of users also offers an opportunity to change the pharmaceutical ecosystem. For instance, Optibrium’s Segall says that these tools “enable easier collaboration through shared project files and datasets, enhancing the efficiency of a drug discovery team.”
If the computational approach is to change the pharmaceutical ecosystem, however, more companies and scientists must adopt and integrate the appropriate computational tools. These tools, Segall says, should be intuitive and easy to use—even for people without a computational research background—and they should have a visual interface. “Related to this,” he adds, “there are many different computational tools that a scientist may use, so it must be easy to move between these, and to move data between programs.”
To address the challenges of adoption and integration, Optibrium developed StarDrop, which Segall describes as an “integrated suite of software for drug
design, optimization, and data analysis.” He asserts that “StarDrop enables scientists to rigorously analyze their data and move seamlessly to the design of the next round of compounds, supported by a comprehensive range of in silico modeling and de novo design capabilities to stimulate the search for new optimization strategies.”

In addition, Optibrium’s Augmented Chemistry suite includes a deep learning platform called Cerella. According to Segall, it “can find hidden opportunities in compound data [and] help guide decisions around which compounds to progress and experiments to prioritize.” Finally, Optibrium’s Inspyra software can facilitate de novo design. Segall says that the software “can dynamically learn from user feedback to automatically generate new compound ideas.”
No simple solutions
Even as the computational tools for drug design improve and expand to more users, the field cannot overcome a fundamental fact, one that Woody Sherman, PhD, CEO at Psivant Therapeutics, states as follows: “Drug discovery is hard, but each project is hard for different reasons.”
In recognition of this fact, Psivant built flexibility into its QUAISAR [QUantum, AI, and Structure-Activity Relationships] computational platform. “One of our distinguishing strengths is the ability to go beyond binding, predicting what happens to the 3D shape of a protein when a molecule binds,” Sherman says. “While we are taught in school that protein structure dictates function, it is really protein dynamics that dictate function, since biology is in constant motion.”
To account for the structural changes that proteins can undergo, Psivant developed a suite of in silico conformational modulation assays that can quantify how binding events impact the conformational states of proteins. “[We use the assay results] to design allosteric inhibitors and agonists,” Sherman explains. “Once we elucidate a novel conformational mechanism, our drug hunters propose design hypotheses that are run through our computational design cycle to efficiently explore chemical space, where we routinely screen billions of virtual compounds in a day, accounting for chemistry synthesizability, ADMET properties, and binding/selectivity.”

Insights gained from QUAISAR can lead scientists to new approaches to drug design. “QUAISAR was initially developed at Silicon Therapeutics, where we designed the first small-molecule STING agonist to enter human clinical trials,” Sherman recalls. “STING is a central regulator of the innate immune system and is challenging for a number of reasons,” including a large polar site that binds endogenous cyclic dinucleotides and the conformation change required for activation.
Sherman and his colleagues at Silicon Therapeutics (which was acquired by Roivant Sciences in 2021) designed their clinical agonist to self-dimerize in the STING binding site. Consequently, two copies of a small molecule with a molecular weight of about 400 fill a binding pocket that usually captures 800 molecular weight cyclic dinucleotides. “When we discovered this class of self-dimerizing molecules, no examples existed of a drug molecule acting in this way,” Sherman notes. “Since we could not rely on existing data or tools, we built tools to solve specific problems.”
At Psivant (which is a Roivant company), Sherman and his colleagues “use QUAISAR continuously in drug discovery projects, leveraging hundreds of in-house GPUs to make decisions fast enough to impact projects,” he says. “Project milestones have been achieved as a direct result of our computational predictions.”
In the future, tools for computational drug design will get faster and handle even more data. As a result, new drugs should reach the market more quickly and economically.
The post Computational Tools Take Advantage of the Data Deluge appeared first on GEN – Genetic Engineering and Biotechnology News.
therapeutics
pharmaceutical
hardware
artificial intelligence
ai
machine learning
software
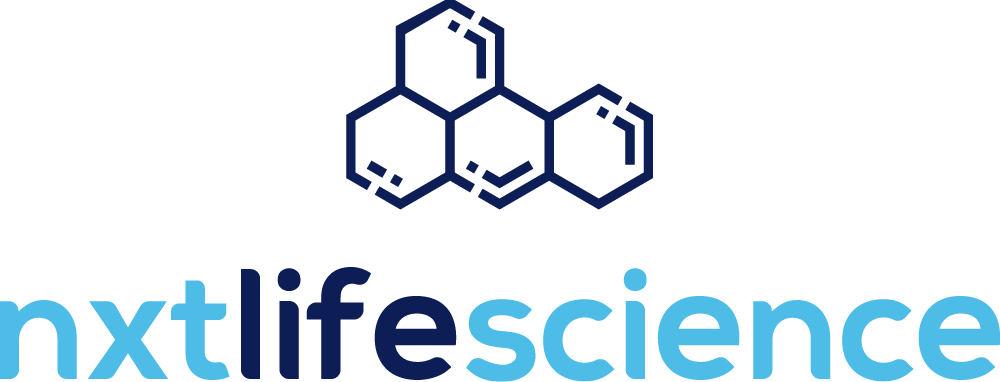
Wittiest stocks:: Avalo Therapeutics Inc (NASDAQ:AVTX 0.00%), Nokia Corp ADR (NYSE:NOK 0.90%)
There are two main reasons why moving averages are useful in forex trading: moving averages help traders define trend recognize changes in trend. Now well…
Spellbinding stocks: LumiraDx Limited (NASDAQ:LMDX 4.62%), Transocean Ltd (NYSE:RIG -2.67%)
There are two main reasons why moving averages are useful in forex trading: moving averages help traders define trend recognize changes in trend. Now well…
Asian Fund for Cancer Research announces Degron Therapeutics as the 2023 BRACE Award Venture Competition Winner
The Asian Fund for Cancer Research (AFCR) is pleased to announce that Degron Therapeutics was selected as the winner of the 2023 BRACE Award Venture Competition….