Life Sciences
Duke professor – a critic of ‘runaway train’ AI development – is named one of the field’s top women
Cynthia Rudin, a professor at Duke University and an outspoken critic of "runaway train" AI development, is one of the top 10 women in the field of artificial…


DURHAM – Cynthia Rudin, a professor at Duke University, is one of the top 10 women in the field of artificial intelligence research and development, reports AI Magazine.
She is “known for her pioneering work in the fields of ML, applied ML, and causal inference. She has also held positions at Columbia, NYU, and MIT,” the magazine says.
Rudin recently was critical of the rapid development taking place in AI, preceding by weeks the issuing of a letter from more than 1,000 scientists, researchers and executives such as Elon Musk and Steve Wozniak calling for a pause in development.
“AI technology right now is like a runaway train and we are trying to chase it on foot. I feel like that because the technology is increasing at a very fast rate. It’s amazing what it can do now compared to even a year or two ago,” she told Eric Ferreri, Senior Writer at Duke Today.
“Misinformation can be generated very, very quickly. Also, recommender systems (that push content to people) in directions we don’t want them to be. And I feel the people haven’t yet had a chance to speak up about this. It’s really technology companies imposing it on us rather than the people getting a chance to decide themselves what they want.”
At Duke Rudin is Earl D. McLean, Jr. Professor of Computer Science, Electrical and Computer Engineering, Statistical Science, Mathematics, and Biostatistics & Bioinformatics PI, Interpretable Machine Learning Lab, according to her Duke bio.
“My research focuses on machine learning tools that help humans make better decisions, mainly interpretable machine learning and its applications,” she says.
“We work on decision trees, sparse linear models and scoring systems, variable importance measures, causal inference methods, interpretable deep learning, dimension reduction, and methods that can incorporate domain-based constraints and other types of domain knowledge into machine learning.
“These techniques are applied to critical societal problems in healthcare, criminal justice and energy grid reliability, as well as to materials science and computer vision. Many of our interpretable machine learning algorithms heavily rely on efficient discrete optimization techniques.”
More about Cynthia Rudin
Artificial intelligence industry is out of control, requires regulation, Duke researcher warns
Duke computer scientist wins ‘Nobel Prize’ worth $1M for artificial intelligence work
The post Duke professor – a critic of ‘runaway train’ AI development – is named one of the field’s top women first appeared on WRAL TechWire.
bioinformatics
healthcare
artificial intelligence
ai
machine learning
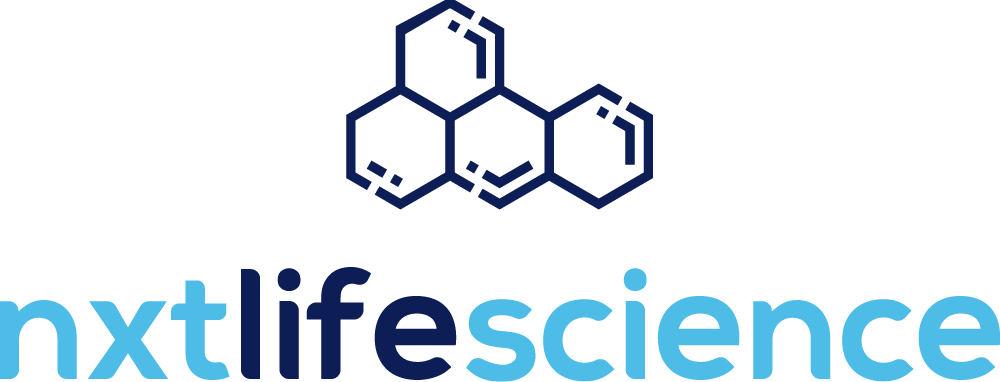
Wittiest stocks:: Avalo Therapeutics Inc (NASDAQ:AVTX 0.00%), Nokia Corp ADR (NYSE:NOK 0.90%)
There are two main reasons why moving averages are useful in forex trading: moving averages help traders define trend recognize changes in trend. Now well…
Spellbinding stocks: LumiraDx Limited (NASDAQ:LMDX 4.62%), Transocean Ltd (NYSE:RIG -2.67%)
There are two main reasons why moving averages are useful in forex trading: moving averages help traders define trend recognize changes in trend. Now well…
Asian Fund for Cancer Research announces Degron Therapeutics as the 2023 BRACE Award Venture Competition Winner
The Asian Fund for Cancer Research (AFCR) is pleased to announce that Degron Therapeutics was selected as the winner of the 2023 BRACE Award Venture Competition….