Digital Health
How AI Can Deliver Benefits in Healthcare Manufacturing and Patient Device Usage
According to Grand View Research, the global artificial intelligence (AI) in the healthcare market size was valued at USD 15.4 billion in 2022 and is…


According to Grand View Research, the global artificial intelligence (AI) in the healthcare market size was valued at USD 15.4 billion in 2022 and is expected to grow at a compound annual growth rate (CAGR) of 37.5% from 2023 to 2030, to reach an estimated USD 208.2 billion. That’s an impressive growth rate, indicating the expected value delivery to a mostly early-phase adoption of AI in the healthcare marketplace. With such aggressive predicted growth, many health industry professionals may ask themselves why they are not investigating AI yet.
AI deploys applications that mimic human-level intelligence, while machine learning (ML) is the subset of those applications that are not explicitly programmed or learned. Together, these rapidly evolving technologies are driving efficiency and productivity across a wide range of industrial manufacturing.
For pharmaceutical and healthcare manufacturers, AI offers deep predictive and data analytics capabilities, and those implementing AI in their manufacturing processes stand to benefit from improved productivity, higher efficiency, and faster production of life-saving drugs or medical devices. The use of AI technology enables, for example, manufacturers to analyze patterns in data sets to understand the implications, benefits, and success rates of new drugs before launching them into the market. Applications such as process modeling enable manufacturers to develop new insights and deliver predictive quality values before measurement information is available, predictive maintenance to eliminate unplanned downtime, and intelligent machine monitoring to eliminate equipment-driven production deviations. Customers can run manufacturing design scenarios and extrapolate from small experimental design cases to optimally determine the next predicted step for more successful test cases. AI, when properly designed, is expected to merge human and machine intelligence to accelerate innovation.
Applications of AI Technology
Imagine equipment essential to producing an in-specification insulin pump (something a large population, and I, rely on). Such equipment is monitored during the production of each pump with classical regulatory measurements and control systems. Today, with modern intelligent AI monitoring, known deviations or anomalies can be detected for any measurements outside normal ranges. Medical device companies know that using and evaluating leading-edge technologies can identify failures earlier within a significantly less costly time frame. This change in technology can dramatically impact manufacturers and end customers.
Modeling predictive control uses mathematical models to drive performance to maximize yields, productivity, and energy efficiency in drying, fermentation, distillation, crystallization, filtration, and other processes familiar to healthcare manufacturing. One relevant focus for healthcare and validation is AI ‘explainability,’ or the ability to understand what drives action and how adaptive/ intelligent applications respond to different conditions. Inexplicable applications seem much less favored in healthcare and life science, as understanding assists AI evaluation and validation. ‘Black box’ closed solutions are less likely to meet the requirements of this set of needs. To be clear, validation is defined as assuring a specific system will consistently meet the requirements and intended use, these are significantly easier to specify and verify compliance with explainable and not black box AI.
Implementation Considerations
Before applications scale to hundreds of use cases in each facility, industrial customers will want to understand the cloud strategy and start to adopt a cloud management infrastructure. While local access has excellent benefits, having shared-cloud management and application control access from the cloud can be essential to provide quick and specialized data science oversite or support whenever needed. A single cloud infrastructure that can monitor and oversee hundreds or thousands of applications simplifies tracking what is working, and what is being used, and identifying any applications that are turned off, failed, or need attention. The cloud can mean a local private cloud or a secure public cloud. Still, the fundamental value proposition is a browser, distributed access to information, and connection of the stakeholder to data to support decision-making. Cloud-based strategies create efficient and distributed environments that can push technology or security updates automatically to many systems. In the IT to OT world that is developing IT system management practices, this will be desired before too long.
This use case inherently takes data, learns to develop a digital twin of the system of interest, and leverages an adaptive digital twin running in parallel to manufacturing to evaluate and support intelligent decision-making.
So, what are some of the use cases that this can lead to in the future?
Many come to mind. Users will be more connected to product maintenance and design teams. The connected world means users who are better informed about their product experiences and motivated to get something more valuable and straightforward in the future, will want to provide medical device manufacturers with feedback on product issues. A direct connection and cloud data store of standard input from many customers can drive early and/or ongoing device updates. This means improved device ownership and more compliant usage, alongside updates that improve user alerts and experiences. This will become important in the early days of, for example, closed-loop blood sugar to insulin pump control, but I do not anticipate that decreasing in the later stages of adoption. Imagine a future where continuous blood glucose monitoring is becoming more and more integrated with insulin pump control. Users currently familiar with adapting their own dosage to carbohydrate intake and activity levels will have “hands-free” adjustments but also discover their own expectations of necessary information levels to be comfortable and be able to accept these new automatic adjustments. As more devices become more connected to the manufacturer, this can promote extended utilization and more active device checkups/check-ins, if needed, from device alerts.
Predictive Maintenance
In both manufacturing and medical facilities, the investment required in equipment is significant. The cost of a high-end MRI machine, for example, can be north of $3M, no small change. On a larger scale, the time and capital investment required to build a pharma manufacturing facility is considerable. Construction can take between 5 and 10 years and cost up to $2B.
The common theme here is that to recoup investment as quickly as possible, optimizing equipment use is key to operational efficiency. More patients can be treated. More devices or therapeutics are produced. That said, equipment failure is a fact of life. Disruptions cost money. Patients must rebook appointments. Manufacturing is delayed.
Remote maintenance has been the accepted model of support for almost three decades. Performance parameters are set and monitored, and deviations are flagged so that technicians can assess necessary maintenance, order replacement parts and so forth. This all takes time, and it’s reactive. As the saying goes, “prevention is better than cure,” and it’s here that AI and Machine Learning (ML) are starting to play an increasingly important role as equipment connectivity to direct monitoring networks moves into the mainstream.
Capital equipment manufacturers are now able to partner with customers, to minimize potential downtime through predictive maintenance. ML learns from past system data and experiences to identify normal and deviated operational patterns which support intelligent predictions with minimal need for human intervention. By collecting enough deep device data AI algorithms can define and model failure modes and predict when a potential failure event becomes more likely. A lot of the investments are being made in collecting data from equipment out in the field and being able to draw insights from that, whether in terms of predictive maintenance, better performance, and efficiencies. The data can be so detailed that equipment manufacturers know where and what components are prone to failure, depending on the usage pattern, and the frequency of use, so they can proactively schedule service calls or advise customers of potential issues, based on that data collection, to ensure action is taken to maximize uptime.
Future Opportunities
Given customer approval, treatment success metrics can be aligned with broader populations, and dosing can be improved via connection to health devices that are becoming more sophisticated. This data can be monitored with duplicate anomaly detection and alert mechanisms used in IT systems to provide early hack detection. In the case of medical impacts, it is personally critical and valuable, however, in the manufacturing plant, sets of AI applications will integrate to provide both local and holistic oversight. The health of the line or plant can be monitored to provide information on decisions such as sanitation or contamination risks, or safety issues when things like systemic anomalies are detected. In addition, production planning and scheduling switchovers can be coordinated and optimized to efficiently manage the site or line activity, maximizing production runs with quality.
It’s still being determined whether these opportunities will happen sooner or later. However, the value achieved and expected from digitizing operations with learning engines and human staff augmentation is exciting. Decision support from helpful, intelligent agents will maximize the value of feedstock, equipment, people, and products.
About Michael Tay
Michael Tay is the Platform Lead at Rockwell Automation, a global leader in digital transformation and industrial automation. Michael’s been working in the area of modeling and AI for more than thirty years with seven patents in the areas of Model Predictive Control and Real-Time Optimization across a variety of industries. His current focus is on democratizing and simplifying successful ML in helping to drive manufacturing performance.
manufacturing
therapeutics
medical
pharma
healthcare
health
pharmaceutical
delivery
device
devices
artificial intelligence
ai
machine learning
data analytics
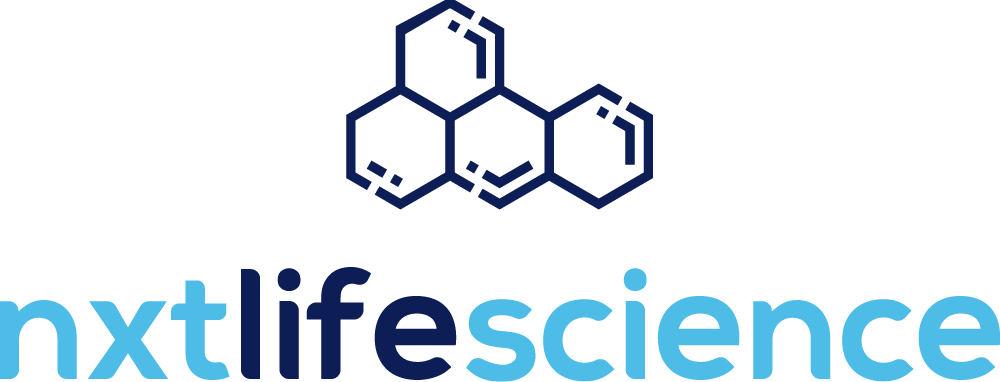
Keep it Short
By KIM BELLARD OK, I admit it: I’m on Facebook. I still use Twitter – whoops, I mean X. I have an Instagram account but don’t think I’ve ever posted….
Asian Fund for Cancer Research announces Degron Therapeutics as the 2023 BRACE Award Venture Competition Winner
The Asian Fund for Cancer Research (AFCR) is pleased to announce that Degron Therapeutics was selected as the winner of the 2023 BRACE Award Venture Competition….
Seattle startup Olamedi building platform to automate health clinic communications
A new Seattle startup led by co-founders with experience in health tech is aiming to automate communication processes for healthcare clinics with its software…