Digital Health
Superluminal Medicines Launches to Drug Protein Conformations in a Flash
A few days after the launch of Superluminal Medicines was announced on August 28, CEO Cony D’Cruz told GEN about the company’s journey, which started as…

Whenever I interview the founders of a new biotech company, I typically inquire about the origin of the company’s name. There is an astonishingly wide range of responses to this question.
A few days after the launch of Superluminal Medicines was announced on August 28, as I was getting ready to speak with CEO Cony D’Cruz, I started trying to connect the company name with G protein-coupled receptors (GPCRs), the targets of their emerging small-molecule drug discovery programs. Everything that sprang to mind was predicated on the idea that a lumen is “the inside space,” be it inside an organ or cell. It turns out that my biologically based assumption was entirely incorrect.

According to D’Cruz, the company’s journey started in January 2022, when RA Capital Management’s principal Nathaniel Brooks Horowitz and senior associate Soumya Ray suggested the name superluminal—it means “faster than the speed of light”—for a top-secret project aimed at tackling drug discovery on a never-before-seen timeline. They considered using a range of computational technologies to expedite each stage of the drug discovery process to accomplish this.
As a result of that idea, Superluminal Medicines has received $33 million in seed funding, with significant contributions from Insight Partners, NVIDIA, and Gaingels, to fuel its drug discovery engine and pipeline of small molecule therapeutics.
A computational crystal ball
In early 2022, the team working on the top-secret “superluminal” project started looking at the landscape of available computational technologies. They brought in tools for protein structure prediction like AlphaFold2 and molecular dynamics simulations based on free energy perturbation methods, which D’Cruz considered useful only with high-quality crystal structures to build a computational model on.
However, D’Cruz said that crystal structures (but not really cryo-EM) have some limitations in the resulting protein construct because the crystal structure is modified to some degree, which can be quite significant when talking about membrane proteins.
“It creates an artificial structure, which is great for getting the crystal structure but not so great for the representation of how it actually behaves in a cell,” said D’Cruz.
They then began to do something that, at face value, seems quite counterintuitive: reduce the sequence homology parameters in AlphaFold2, which essentially works by finding similar sequences to the input, extracting the information using an especially neural network architecture, and then passing that information to another neural network that produces a structure.
“If you give AlphaFold2 a sequence, it goes out into the ether and picks everything that’s structurally available that looks like that sequence,” said D’Cruz. “If you start reducing the sequence homology constraints, you basically draw a wider set of structures and start seeing something very interesting—protein flexibility. You get this dynamic movement of the proteins computationally because you can stitch together all the various conformations.”
Cryo-EM at scale is challenging, expensive, and time-consuming, but D’Cruz and his team are trying to do just that with computation to optimize drug discovery cycles.
With this as their starting point, the top-secret project morphed into the stealth company Superluminal Medicines and a team began predicting complex protein structures.
By using these protein structure prediction tools, biologist-turned-co-founder and head of discovery Ajay Yekkirala, PhD, began considering what would happen if you could target particular conformations of a complex protein, like GPCRs, to elicit specific downstream signaling. A year ago, they started to see some encouraging indications that they could predict conformations of a specific GPCR.
Protein nonconformists
The next piece of the computation pipeline puzzle was to connect different GPCR conformations to different signaling pathways. This gave them a place to start their virtual screening for small molecules that work only with certain GPCR conformations.
According to D’Cruz, the fact that so many drugs fail in the preclinical and clinical testing stages due to toxicity events has a significant impact on this step. Superluminal has tried to get ahead of toxicity events by trying to understand as much as possible in terms of Absorption, Distribution, Metabolism, and Excretion (ADME) liabilities, spending significant resources on building a predictive tool specifically for this.
While D’Cruz said it’s too early to say how well this toxicity prediction tool works, at least it provides their medicinal chemists with a line of sight of what’s possible in terms of an issue downstream that they can then start thinking about and avoiding by design.
D’Cruz, who spent more than nine years at Schrödinger, says that there is no way that Superluminal can or will skip any testing that’s traditionally involved in drug development and discovery. Where these tools will have a major impact is in minimizing animal testing by making predictions upfront and only testing limited sets of compounds to ultimately speed up and optimize the drug discovery cycle.
I pressed D’Cruz on the limits of their computational approach, asking if there was any value in getting their own crystal structure and CryoEM data. For this, Superluminal initially teamed up with Thermo Fisher and The Cambridge Pharmaceutical Cryo-EM Consortium. Now, Thermo Fisher has put out quite a few CryoEM microscopes, allowing Superluminal to expand the number of sources of structural protein data.
“We’re always going to need structural validation to finally get to the ground truth,” said D’Cruz. “However, we don’t wait for it in order to progress a program. So the definitive go-or-no-go is actually in the cell. Can we elicit the appropriate biological response we want? If that’s the case, then we move forward. We always try and get a structure, but we don’t wait for it.”
Hypothetical pudding
The targeting of GPCRs has been at the heart of several recent company launches in the past few years, notably Septerna, which was launched with the backing of Nobel laureate Robert Lefkowitz, MD. Earlier this month, Solu Therapeutics launched behind its CyTaC (Cytotoxicity Targeting Chimera) platform to drug cell surface receptors, including GPCRs.
Superluminal’s strategy is more akin to that at Solu Therapeutics. D’Cruz said that while this funding round will help Superluminal develop a proof of concept that creates value through targeting GPCRs, their computation is by no means limited to working with GPCRs.
After all, the initial top-secret superluminal project started off focusing on not only GPCRs but also enzymes. D’Cruz said that one of the first proof-of-concept examples of their computation focuses on an enzyme that has evaded crystallographers for years.
“It is an enzyme that we chose to go after because, for years, we couldn’t get the crystal structure appropriately because the five-prime end was capped,” said D’Cruz. “We predicted the structure without the cap and docked compounds, and then we got a crystal structure without the cap, proving that we got the prediction correct. So, we know we can do enzymes.”
According to D’Cruz, Superluminal’s choice to target GPCRs was purely a “business decision.” In the future, D’Cruz hopes that they will be able to partner and expand the domain of applicability of the platform beyond GPCRs.
What D’Cruz thinks will help Superluminal Medicines stand out from the rest of the pack pursuing GPCRs is the quality of his team. The team currently consists of ten people, which includes experimental biology scientists in Yekkirala, who was a postdoc at Harvard Medical School and Boston Children’s Hospital before co-founding Blue Therapeutics and then ending up as a VP and the head of biology at RA Capital Management. Another key figure is Yamina Berchiche, PhD, who previously was at the Institute for Protein Innovation and goes by the name “Dr. GPCR.”
Additionally, they have brought in experienced computational scientists, such as head of engineering Yang-Ming Zhu, PhD. Zhu spent almost two decades with Philips, reconstructing 2D positron emission tomography (PET) scans into 3D images for diagnostics, and is, according to D’Cruz, Superluminal’s “machine learning guy who is used to dealing with complex and difficult data sets and brings a different way of thinking to drug discovery and development.”
To impress upon me the importance of out-of-the-box thinking when it comes to computation, D’Cruz gives an anecdote from last year, in which shortly after joining, Zhu asked if there was any money for IP. Zhu had already developed several proprietary machine learning algorithms by taking algorithms from different applications and industries like manufacturing and transportation, which have high-data complexity, and applying them to their drug discovery pipeline.
“We like to fool ourselves in biology, but the data is actually not that deep—we don’t have a lot of data,” said D’Cruz. “That’s one of the limitations of using machine learning in drug discovery and development. There are far more pictures and data for cats than there are drug discovery programs or even compounds. So, [Zhu] managed to bring in new ways of thinking to our industry, which is what we’re looking for.”
With this initial team and funding round, D’Cruz emphasized that Superluminal is focused on developing assets.
“Obviously, we spend money developing the platform, but in service of the assets,” said D’Cruz. “We try and understand the biology problems, the fundamental things that we’re trying to do once we identify the targets, and then the tool sets we need with either what’s currently available or what we need to develop to get to that point. At the end of the day, it’s the value of what we create that anyone’s going to judge us by. It’s really hard to value an algorithm.”
In the growing space of AI-based drug discovery, no one says that their algorithms are average—they say that they’re the next best thing. While confidence, to some degree, is necessary to move a project forward, the field is not at the stage where there is an answer for the best approach or for the ones that may be wildly wrong—even dangerous. With such a fast-growing space, the only way to judge a company’s value will be through robust and validated assets. The proof of the pudding is in the eating, not the dreaming.
The post Superluminal Medicines Launches to Drug Protein Conformations in a Flash appeared first on GEN – Genetic Engineering and Biotechnology News.
manufacturing
diagnostics
therapeutics
small molecules
medical
biotech
ai
machine learning
hospital
pharmaceutical
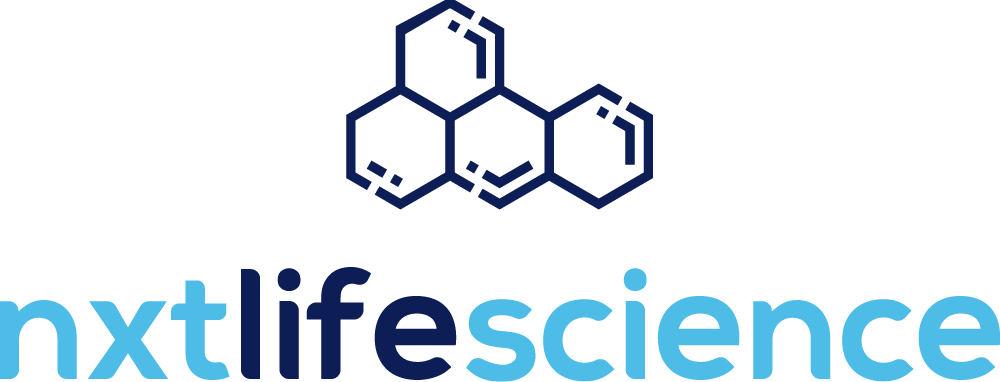
Keep it Short
By KIM BELLARD OK, I admit it: I’m on Facebook. I still use Twitter – whoops, I mean X. I have an Instagram account but don’t think I’ve ever posted….
Asian Fund for Cancer Research announces Degron Therapeutics as the 2023 BRACE Award Venture Competition Winner
The Asian Fund for Cancer Research (AFCR) is pleased to announce that Degron Therapeutics was selected as the winner of the 2023 BRACE Award Venture Competition….
Seattle startup Olamedi building platform to automate health clinic communications
A new Seattle startup led by co-founders with experience in health tech is aiming to automate communication processes for healthcare clinics with its software…